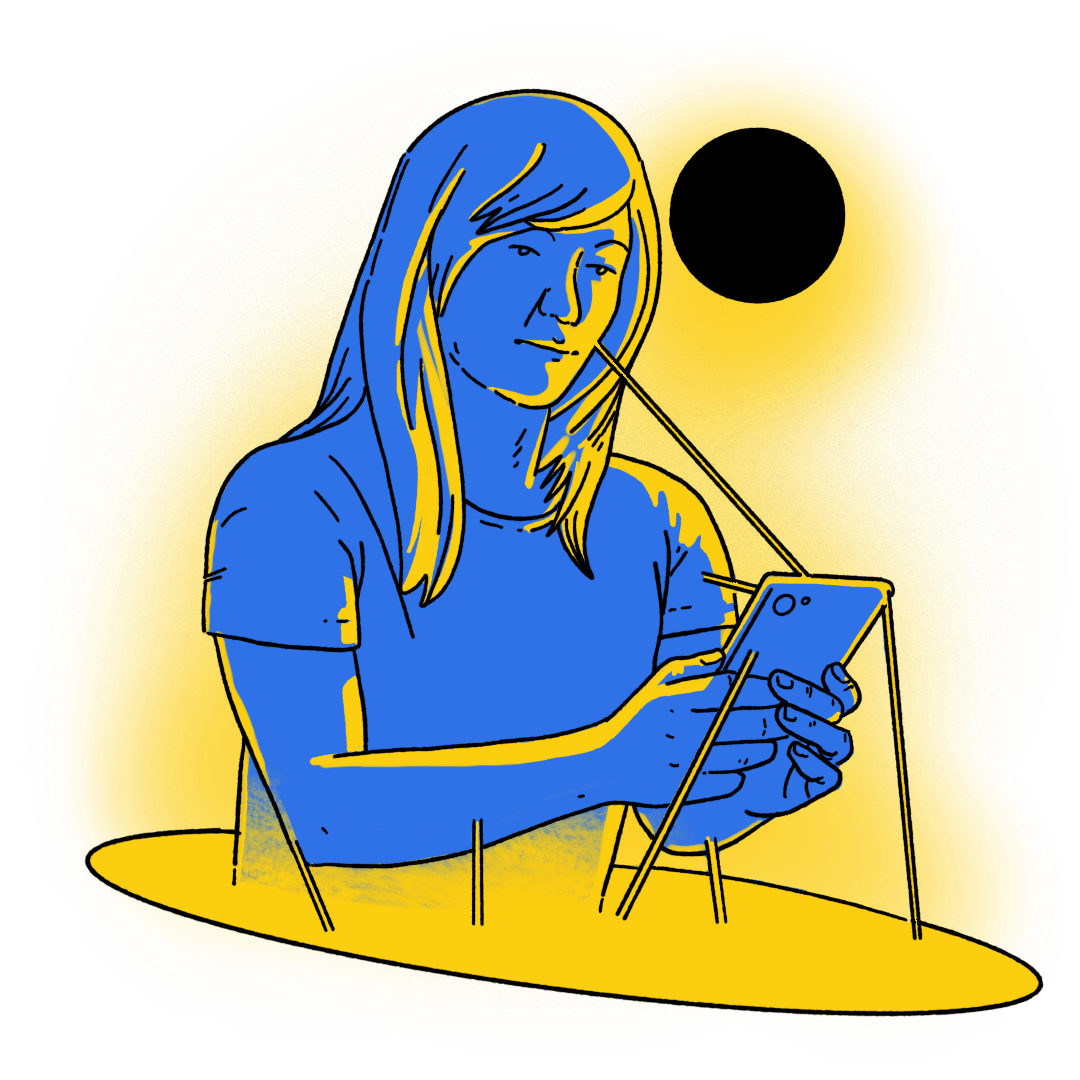
Summer usually brings everyone's rhythm a bit down but rest assured, governments are busy figuring out how to reign in Big Tech's overwhelming grip over our everyday lives. Things are in motion and have been for a while now..
On this issue we are joined by Pedro Bizarro, cofounder and Chief Science Officer at Feedzai, to discuss responsible A.I., the evolution of the Portuguese startup scene, the struggle of hiring and the future of the country's entrepreneurial ecosystem.
- Lawrence
G7 leaders reached an "historic" agreement to tackle tax abuses by internet giants and to introduce a global minimum corporate tax rate of 15 percent.
European consumer lobby group is backing the E.U.'s antitrust case against Apple, which alleges it distorts competition in the music streaming market while E.U. antitrust officials started investigating Google's ads business. Germany's Federal Cartel Office is getting busy having launched proceedings against Apple over pre-installed apps and in-app purchase system, as well as against Google's News Showcase on whether it hinders competition.
The U.K. is not staying behind with the Competition and Markets Authority which started an investigation into Apple and Google over their dominant position in the mobile phone market as well as opening a probe against Google and Amazon over fake reviews.
In the U.S. top antitrust lawmakers introduced a legislative package that could overhaul the nation's antitrust laws in an attempt to rein in the power of Amazon, Apple, Facebook and Google. Also, Democrats and Republicans came together to confirm Lina Khan to be chair of the Federal Trade Commission.
Russia fined Facebook and Telegram for unlawful content. Nigeria suspended Twitter "indefinitely" after the platform removed a post from the president. The standoff between the Indian government and Twitter escalated after accusing the social media giant of not complying with local laws.
Amazon settled for $61.7m over pocketing driver tips but is facing a possible €425m fine in the E.U. related to alleged violations of Europe's General Data Protection Regulation.
Google said it will adapt its ad technology after France delivered a $267m fine, making it easier for competitors to use its ad tools.
A Texas Supreme Court ruled that Facebook could be held liable if sex traffickers use the platform to prey on children.
Since 2018, Amazon Web Services has hired at least 66 former government officials, most directly from government posts and more than half from the Defense Department.
Apple's new privacy feature, designed to mask users' internet browsing, won't be available in China.
Facebook has suspended ex-U.S. president Trump for 2 years
Bing temporarily censored image searches for 'Tank Man', even in the U.S.
YouTube blocked videos from a human rights group of testimonials about missing Uyghurs in China as they contained ID cards.
"Experts Doubt Ethical AI Design Will Be Broadly Adopted as the Norm Within the Next Decade" by Pew Research Center
"Collective data rights can stop big tech from obliterating privacy" by Martin Tisne
"Algorithms already fire and 'give' loans. How to make them fair" (interview with Pedro Saleiro from Feedzai, in Portuguese) by João Tomé
"A Homeless Amazon Warehouse Worker in New York City Tells Her Story" by Lauren Kaori Gurley
To get these delivered to your inbox, subscribe to CFT's monthly newsletter at the end of the page.
Lawrence - Welcome! For this issue we have the pleasure of being joined by Pedro Bizarro.
Pedro is one of the co-founders and the Chief Science Officer of Feedzai, where he has helped develop the company's industry-leading artificial intelligence platform to fight fraud.
Amongst other things he's worked for CERN - the European Organization for Nuclear Research. He's been an official member of the Forbes technology council, a visiting professor at Carnegie Mellon university and I could go on but I think that gives a pretty good picture of who we are talking with today. So welcome Pedro and thanks for being here with us.
Pedro - Thank you, thank you. My pleasure. Thanks for inviting me.
L So I'm really happy to have you here because this project, as we discussed last time, is about giving visibility to great examples that are acting in everything that is ethical and responsible AI, which is precisely what I want to talk about with you.
So to set the stage for listeners that are not familiar with Feedzai, briefly, Feedzai is a company that provides financial crime detection services to financial institutions using big data and artificial intelligence.
P That's right. So we work with large financial institutions, large banks and payment processors and also we have large merchants.
L You have been one of the companies in Portugal that has been talking more visibly about responsible AI. That is definitely an important topic for you guys at Feedzai. This topic is new into the mainstream conversation I would say. Over the past five, six years, it's been heavily more discussed. So it looks that you guys in a way have been ahead of the curve, at least in Portugal, talking about this and worrying about this. And I'm very curious to understand why that's the case. Can you tell us how that came to be?
P Well it's that old sentence "with great power comes great responsibility". And I do feel that AI is a great power and is being used almost always for good, in a lot of use cases throughout our lives. Sometimes we don't even realize we are using AI. It could be a Google search, an Amazon recommendation, Netflix, Spotify, basically all the major services that we use today are using AI in the background. Even a job search on LinkedIn and so on.
So in about 2016, when I read the now famous book "Weapons of Math Destruction" by Cathy O'Neil, it was the first time that it really sank in for me that you can develop really good machine learning models according to some business goal, but without you realizing you can produce some very nasty side effects that can affect people in their daily lives.
Sometimes the people are not aware, the companies are not aware. Even the data scientists that were designing the systems, sometimes they didn't have any bad intentions, but they were not even aware.
That was really the first time that I realized this can happen basically anywhere because we can introduce bias by lots of decisions with choices of data sets, sampling strategies, decisions of what to do when there's missing data, model parameters. So there's a whole number of decisions that you make when building a model and some of those can produce biased or unfair results where the model is hurting a group more than others or reducing the likelihood of detection for example.
And not only it can hurt in that specific case but, even more concerning, sometimes these could create feedback loops where the model is affecting the reality and the reality is affecting the model and so on. So you keep on making it worse over time.
So that's when we started at Feedzai looking at responsible AI. We were already, even before that, looking at concerns of model explainability, how to explain model decisions to users. That was already a typical concern in financial institutions, because sometimes as a model that is deciding if you allow an account to be opened or not, if you block a card or not, and then you want to either explain that to the end-user or internally to a data scientist or even to an external person like a regulator.
So there was already a need for explaining, but it was not until then that I realized that besides the need to explain, there were other more complex needs about the responsible use of AI.
L So being transparent is part of being responsible, right? Being able to account for why the algorithm decided X instead of Y, right? Because someone is going to ask "why can't I have access to this insurance or this loan or this service and the other can?".
So, the term responsible or ethical, you know - I'm going to ask a tougher question now - can be vague, right? It can be interpreted in many ways. So I'm curious to know how you interpret it at Feedzai?
P That's I think a great point. You are very right. Responsible is a broad term and I'm pretty sure that if you ask 20 different people you have 20 different answers. In my perspective responsible AI is one of those umbrella terms that includes many things inside of it and for me it includes at least the following.
We are developing responsible AI systems if first they are fair so they are not hurting one group more than others. So it's really about the disparate impact. Are we impacting these groups more than another? That is one component of responsible AI which is having less biased or unbiased models.
Another component of responsible AI has to do with expandability. Do we understand the model? Do we trust the model? There's many times a human in the loop and the human in the loop can trust or not the model and sometimes they are trusting too much. For example, computer say so, so if the computer says so I'll press it [the button] and sometimes they shouldn't and vice versa.
Sometimes they are not trusting the model and they should trust the model. So there is a huge component of how we the users, the humans in the loop, trust or not the model. How are they understanding the model decisions (if they are understanding the model decisions)? So those components also play a role.
So the first one was fair model, the second one has to do with expandability and trust.
A third point for me of responsible AI has to do also with what nowadays is called MLOps: machine learning operations. Maybe not many people include these within responsible AI but I also include it in the sense that, for example, models can degrade over time by concept drift, by data drift, if you're using an anniversary or if your data center has less resources or more resources.
What happens is that in reality, the world is changing and that causes the model to also change its performance. So I think it's a more responsible use of AI if, when the model degrades, you discover that. You find out that the model degrades and you automatically retrain the model or raise some sort of alert for people to retrain or to find out that something is incorrect.
For me that is also a dimension of responsibility. How to adjust to the changing world and also how to adjust to reasonable resource uses?
We know that there's a big concern with modules that are too expensive to train that they are taking thousands, sometimes tens of thousands of machines to train so their energy consumption is too high sometimes for the benefit that they bring.
L Yeah, you're pondering the trade-offs regarding the gains. The effort and the drawbacks in terms of energy? That's a good point, yeah.
P And maybe a fourth dimension of responsible AI is so not only the model must be fair and explainable and automatically adjust to the changing world, but it must also be used for good right?
You can have a fair model that hurts men and women in the same way, but hurts both of them, right? Maybe you have a model that is manipulating people into buying something or to adapt or something like that.
So not only the model itself should be less biased and unexplainable and use a good amount of resources but what you do with it is of course also a big part of the responsible AI perspective.
Those are the four big dimensions of responsible AI.
L Right. You mentioned something which also is one of the things that I want to talk with you to the extent that you can talk about it, which is, as you said, very well put: it is a moving target. You will never reach it and then that's done, like "we have achieved fairness". You need to constantly tweak it. And so you need to understand, as you said, whether or not something should be tweaked? Is it outside of the model that we deem being fair, being right?
Have you guys developed anything like, you mentioned alarms. Have you developed something that tells you something is going off track?
P Yes, so we have a number of people working on what we call "auto everything". In reality, they are working in what's now called MLOps. We've been developing tools to do what we call model monitoring and feature monitoring.
So for example we are monitoring how the distributions of the scores of the model are changing over time? So you expect some distribution of scores so you train your model, you measure the distribution of scores and then in runtime you can keep track of that distribution of scores and you can realize: "Oh!, something is very off here. My distribution of scores is way off compared to what I was expecting". So that's one thing we do. We are automatically monitoring the model. And that was our first step.
We are also working on what we call feature monitoring. So one thing is monitoring the models: the end results, the decision of the model. But the other one is monitoring the input to the model. So the features that go into the model. And that's slightly more complex because first there's hundreds of them. So not just monitoring the final decision, but you are monitoring hundreds or thousands of features. And if there are many features, it's likely that any one of them is maybe off for some reason.
We already developed even patent pending work on feature monitoring and not only on the part of detecting that the features change, but also that efficient part. How can you do that efficiently? Because in production, you are having thousands of transactions per second, each one with hundreds of features and each one needs to be processed in a few milliseconds.
How are you computing all of those distributions efficiently and keeping track of that? And also from a statistical perspective, because there are so many features and it's likely that a few of them are off for some reason. You don't want to raise too many false alerts, so how do you statistically decide when it's really time to realize that something changed and to raise an alarm?
And also from a user interface perspective. How will you monitor a stream of data with 500 features? You cannot show 500 features so you need to be smart in how to show alerts and allow people to drill down into the alert and then identify the features that are changing and what's going on now compared with what normally should be going on. And what were the records of the instances that caused that change and so on? We are working in all of those areas.
L Very interesting. So let me get back a bit just before. You became aware that with great power comes great responsibility. This is something that touched you. And then you went into an all hands meeting and you said: "guys from now on, let's be fair". You know? Like "let's bake fairness into our day-to-day work" right?
I don't imagine that was how it happened, but first of all, do you have just a nucleus of people that are focused on that? And then the rest of the company is basically " unaware", let's say? Or is it something that comes often on a day to day conversation across teams?
And also you have roles besides pure engineers? Do you also have other backgrounds that can also influence how you think about the models and how you think about controlling for bias and fairness? It's a big question.
P It's like two or three questions, but let me go. I think the first one, which is how did we start working on these operationally in terms, if it was like an all hands meeting and then I started pushing.
So I approached it as I approach almost all new things, which is first I was going to learn about the subjects. So I spent to be honest, a few years, initially just reading, reading, understanding, understanding the state of the art within reading books, within papers, getting to know the research of the top institutions from academia side, from industry side, what they were doing, what they were not doing, what were the opportunities and what were the risks, what type of explanations were there and so on.
And then I was able to identify that "okay, this makes sense". So in my mind, first I was trying to understand: is the problem complex? Is the problem going to appear on our domain? Is the problem relevant for our clients and for our data scientists?
I concluded that yes, that it was unavoidable. I saw it affecting other use cases, other companies in health at first and in law also. Very famous examples for Amazon and Goldman Sachs and other companies with explainability issues and say: "okay, we need to address it". And if we're going to do it, let's do it right.
So I assembled a team to just work on this and they are called internally F.A.T.E., which stands for Fairness, Accountability, Transparency, and Ethics. We created a team from scratch to just focus on this. And initially I also spent a couple of years so as you can see, this is a multi-year effort, right? We started working on this late 2016. They spent a couple of years investing in tools and algorithms and trying to improve things because we realized that in our domain, if you have a good idea, but if you cannot put it in production it doesn't matter.
For me, the question was not only finding good techniques to avoid bias or good techniques to detect bias or produce explanations. It's: can we actually put these in the day-to-day pipeline of data scientists? Can you put this in production? And that's really the challenge. Are these things easy enough to use, fast enough to use that a data scientist under pressure, developing a model for a client with lots of deadlines, lots of constraints: is she going to use the tools that allow to develop fair models? Because if the tools are not easy and good to use, they are not going to use them. So we really spent a lot of time combining the research side of bias detection and explainability and so on with the engineering side of how to put these in the day-to-day usage of a data scientist.
So that was the first part of your question. The second part is if this is a single team working on this or more people are working on this. So initially it was a single team, a team of about five people, but now their impact grew to basically the entire company.
The tools started to be used by other people, we have many training sessions internally with people from the engineering side, product side, customer success side, marketing and sales. We have blog posts, we have different materials, websites. As everything that makes sense, it starts small and then it grows to impact many teams and actually the company at the global scale. And it has been presented to our end clients, to analysts, external analysts that are analyzing companies like ours and competitors. Now it's a global thing in the company.
L That's great. I would like to be like a spy, just like looking at people because in a way you need to change a little bit the paradigm I guess of an engineer that is enticed by a complex problem and you're adding a somehow abstract layer of complexity on top of something that is already hard, right?
In a way you are approaching him and saying: "besides these things, you also need to account for this set of other things", which are still relatively fuzzy. We're still trying to figure out what those things are concretely so that we can push them into production, as you were saying.
And I'm trying to think what were some sort of reactions or the feedback, how was the adoption of it you know?
P I think it was fantastic to be honest. I think because we also have a very strong engineering culture, even the data science team, the research team. We are also users of our own technology so we know that whatever we need to develop must be easy and must be feasible for the data scientists and users.
And I think at the end of the day, our data scientists and any data scientists, they really want to do the right thing. So we didn't have a single case of a person saying: "oh, I don't want to do that". Not a single person.
Everybody was excited. Everybody was: "okay I'm so glad you guys are doing that. This is a piece of mind. It takes some weight off of my shoulders. It allows me to feel good with myself and to feel good with my work". So everybody was seeing these concerns with responsible AI and bias, the famous movie now Coded Bias, right?
Everybody was seeing these things happening out there. Even our clients were seeing that, our CEO was seeing those concerns. So when we developed the ability for people to do that, there was actually a sense of relief that: "okay, we are doing it well, we are doing the right thing". And the other part of it was also I think, positive feedback, because we felt that we are ahead of the state of the art.
So that is also good, right? You're doing the right thing and you are ahead of the market. So those two things. made it that the internal feedback and external feedback was also very, very positive because it's like a win-win: you're ahead of the market and you're doing the right thing and at the end of the day, everybody benefits. The company benefits, the internal developers benefit and external clients also benefit. So it's a win-win-win situation. Very positive feedback.
L And I'm guessing, just to add on what you said, that in terms of values, in terms of the impact of the company, I guess that any person is happier to know that they're just not building just the product. Period. Right? They are doing it in a sort of mindful way in terms of how it will affect the client and the market and the users of it.
P And we were also lucky to win a number of awards worldwide with our responsible AI work. Our algorithm Fairband got four or five different awards, like a FinTech breakthrough award, we were featured on Fast Company on the software side and as an honorable mention on the AI side. So we felt that it was not something that we were seeing only us like the market was recognizing these developments, these algorithms, these ideas as valuable and giving us awards for that.
So everybody felt really like it was a win for the entire company to have started investing in this so long ago, because now it was giving dividends.
L Yeah, for sure. Like concrete not only with clients, but the market recognition that it's a tendency that is valued.
One of the things that I also asked you is the composition of the teams, right? I have discussed with people (see CFT Issue #3) that argue that if you only have engineers then you may not reach the best solution, let's say, because you're missing some other points of views or ways of looking at things.
Do you guys only have engineers or do you have other consultants or other people that help you look at things differently regarding ethics and responsible AI?
P So on the engineering side, we actually have a very varied set of people. We have people from physics background, math, statistics, but also biomedical engineers, computer scientists, so all sorts of backgrounds which is interesting.
But we also work a lot with our internal team of lawyers and legal people that have a concern on how to describe this, what is the impact in terms of law and regulation and also with the marketing team and the sales team. So it's not just the research team that is involved. Many people outside the research team are involved. Even in the research team we have the typical data scientists, but we also have data engineers and visualization engineers.
So it's not only data scientists that work in this subject.
L Awesome, that's interesting. So one thing, which is, how easy has it been to assemble your team over the years? I know you guys are already a considerable amount of data scientists. For our market in Portugal I think it's pretty considerable.
So how easy or how hard has it been in terms of finding those individuals?
P It's hard. It's hard. So I think hiring has probably been the top one challenge. Almost always since the beginning. As I say, we are always hiring, even when we are not hiring, because it takes so long to find good people in terms of their background and experience and mindset and culture.
And we are always looking for people. We are always doing interviews. We are always trying to select, always trying to grow the team. And the market is not gigantic. We feel that there are not enough people with all the background that we would like to have or with all the experience that we would like to have.
It's surely a challenge. I think it's not a challenge just for us, I think it's a challenge across the world. I realize that the universities are now offering some degrees that they were not offering a few years ago. Master's in data science. Almost all major universities are offering that, which was not the case five years ago. We feel that there are more people in the job market and even people that were not data scientists they kind of learned new techniques and became data scientists as well. But it's still a challenge, yes. Probably one of the biggest challenges is hiring.
L Yeah. Well, we are a small country compared to other countries that produce a lot of engineers, Ukraine, for instance.
P At Feedzai we have, I read some statistics, the other day, we have 47- 48 different nationalities in the company. So we hire from lots of different countries. Of course being based in Portugal the majority is still Portuguese, the ones in Portugal, but there are many people from the Eastern European side, from Brazil, from Asian countries. So it's not just Portuguese. But it's true. It's hard to hire in the market, yes.
L I'm using this question to segue a little bit into some of the things that we spoke the first time, which is about would it be possible for us in terms of a country to find this sort of niche? All right, let's be very good at producing and generating people that have this mindset, the mindset of building responsible AI, doing great machine learning models that account for fairness, account for societal issues.
Would that be something that you believe is possible for us in our reality, or are you guys an outlier?
P I totally believe it's possible. I totally believe that it is possible for a small country like Portugal to be a leading voice in a specific area.
For example you know that New Zealand is a world leader in rugby, right? Or Iceland in music or Israel in cybersecurity, right? Even small countries, they can be a reference in whatever area they choose if they really invest. For instance we are also very good at soccer for the size that we are, we are strangely good at soccer. But why is that? It's because we have been investing for literally decades in youth schools for soccer teams and coaches.
And so the investment is really nationwide and across generations. And I think if we keep on investing in a specific area we can be good in any area that we decide to be good at. I remember when we were first raising money in Portugal twelve years ago - and I also told you this, when we talked the other day - we went to a number of U.S. investors, and I remember at the time they were asking us: "you are a Portuguese this company? What big Portuguese exits have been there? What is the history of the country in terms of startups and tech?".
For them it was strange. Who are these guys coming from this country that is famous for Fado and football and "pastel de nata" and now trying to sell a high-tech company?
But they were sort of right. I remember at the time the biggest exits that we had in Portugal were two or three companies that altogether they sold for like $200m.
But now if you fast forward 12 years, right? We have Farfetch being valued at $18b or so, OutSystems $10b, Talkdesk $3b or $4b. Not even talking about Feedzai, there are dozens of companies here today, DefinedCrowd and Unbabel and so many companies that are valued already at hundreds of millions of dollars and they can potentially easily reach billions of dollars. And what happened? What happened was time essentially, right? 12 years since then, but also a strong investment in multiple areas: startups but also the startup ecosystem, the VC money, the founders.
Of course there's plenty to be done still, but the picture here, today in 2021 in Portugal, is radically different from 2010. Is radically different. Now we have big exits that we can show in our sleeves to the world, right? We have good examples, we have good engineering. You have companies that are worldwide leaders in their specific domain. OutSystems is a worldwide leader in their domain. TalkDesk as well. Feedzai is also recognized as a leader in fraud detection.
So I think it's completely possible, but we need to continue on investing in multiple areas and education on the university side. Building the ecosystems, all the startup places of incubation that we are having across the country that didn't exist 10 years ago. All of that creates this ecosystem, just like the youth schools of football, right? We need to have lots of little startup incubators. And some will die, most will die, but many people will learn and will create a culture of entrepreneurship and investment and risk-taking and changing the world for the better.
If we keep on investing, why not Portugal being a leader in responsible AI? I totally think it's possible. And right now I think we are in a good position worldwide. The field is hot, it's just starting. There's a lot of research, but there is not yet lots of applied products, really being responsible in the way that they were designed from scratch like that. So I think that the opportunity is here for the taking and I think we should invest in it.
L I appreciate your answer. It's true that when you look back, it's been an incredible leap in so many ways in what entrepreneurship is risk-taking, as you were saying.
I believe Portuguese are a bit risk averse people by nature, at least that's the idea that I have, but things have been changing. There's one thing that you mentioned, which is the ecosystem, a lot of bright engineers, a lot of bright people, scientists.. The market wasn't able to absorb them, right? You didn't have Feedzais or Unbabels or bigger companies that would justify the existence of those people in our territory. And so they just go to the U.S., France, Berlin, whatever, and you lose those assets that can create new companies and create those new categories.
So it's a whole as you said. To finalize, investing in education, but what else? Like what is some stuff that we should be paying attention to as a country or is the ecosystem good enough? Should we have more mechanisms? Should we have other ways of approaching it in your opinion?
P Yes. But before I answer that, let me just touch briefly. You mentioned that there's some brain drain that we are losing people too.
L Well, we were more now I would not be as sure of that.
P And that I think we still lose some but it can actually be beneficial, right? Sometimes if people go outside and learn and go to top companies and learn and become better scientists, better engineers, even if they don't come back, they build a good reputation or they start a company in the U.S. for example and they have a local office in Portugal.
I've seen that happen. For example, many people in India went to the U.S. and then one generation later, many of them returned to India and started Microsoft research labs and Oracle and IBM research labs in India.
If you look at that with a perspective of 10-20 years, maybe that's not too bad. Right? We have amazing professors that graduated from Portugal and went and became top professors worldwide. We have the same with engineers, for example, Diogo Monica was an engineer in Portugal, went to Square now and he's starting his own company Anchorage, which is doing great. And I think it's possible for people to go outside and come back one way or another, maybe in a few years, maybe in 10 years, maybe as a cyber organization. But I think that the net impact can still be positive in the longer run.
And now going back to the second part of your question, what can we do in Portugal to improve things even more?
So one thing is to make Portugal more appealing to entrepreneurs and to those Portuguese abroad to come, but also to other entrepreneurs to come. Our law is still too complex. Our taxes are still too high, many things that are common in startups like stock options and things like that are not easily translatable in our law. They are hard to execute.
So there's an amount of taxation and regulation that makes it hard for a founder to start a company in Portugal or for a person to join a startup in Portugal. And I think we can reduce that barrier of entry if we make it more appealing from that perspective, from the legal perspective, from the taxation perspective.
L Yeah. The legal system must be clearer for investors to navigate and they want some expectability and conflict resolution not to be hard.
P But that is in addition to what you also said: continuing investment in innovation and education and research. I think we should invest way more in our universities, we should finance more our professors and students. We should have more scholarships and more collaboration.
Education is really the future of a country and I think we should put more money there.
L I'm a hundred percent behind you on that. And on that note I'm going to ask you, do you want to leave any message for our listeners or anything that we should be on the lookout for that Feedzai is working on or just anything that you want to throw out there?
P So we are doing a lot of work on responsible AI, on algorithms that are able to not only find good models, but find good models that are responsible, but we also invest a lot in visualization, novel machine learning techniques and in active learning.
So you should be on the lookout. Keep track of my posts on Twitter and LinkedIn, I'm always posting about the development that we are doing, the papers accepted, the papers on arXiv. We are likely going to start a YouTube channel with our own presentations - research presentations.
So we are really trying to share our state-of-the-art to the world to inspire others and to help others to also benefit from the research that we're doing. So that's why we've been publishing a lot on conferences, arXiv and now on this new YouTube channel that is upcoming.
L I'll be on the lookout. I will link your profiles in the transcript and everyone go follow Pedro. I've been following him for a bit, he publishes interesting content.
Pedro, thank you so much for being here. I really enjoyed this conversation and listening to how Feedzai does things. It's super exciting to see this happening in Portugal. And again, thank you for sharing all of that with us.
P My pleasure, it was a lot of fun.
L I'm sure we'll be talking again. Take care.
P Take care, have fun. Bye bye, thanks.
L Bye.
If you've enjoyed this publication, consider subscribing to CFT's monthly newsletter to get this content delivered to your inbox.