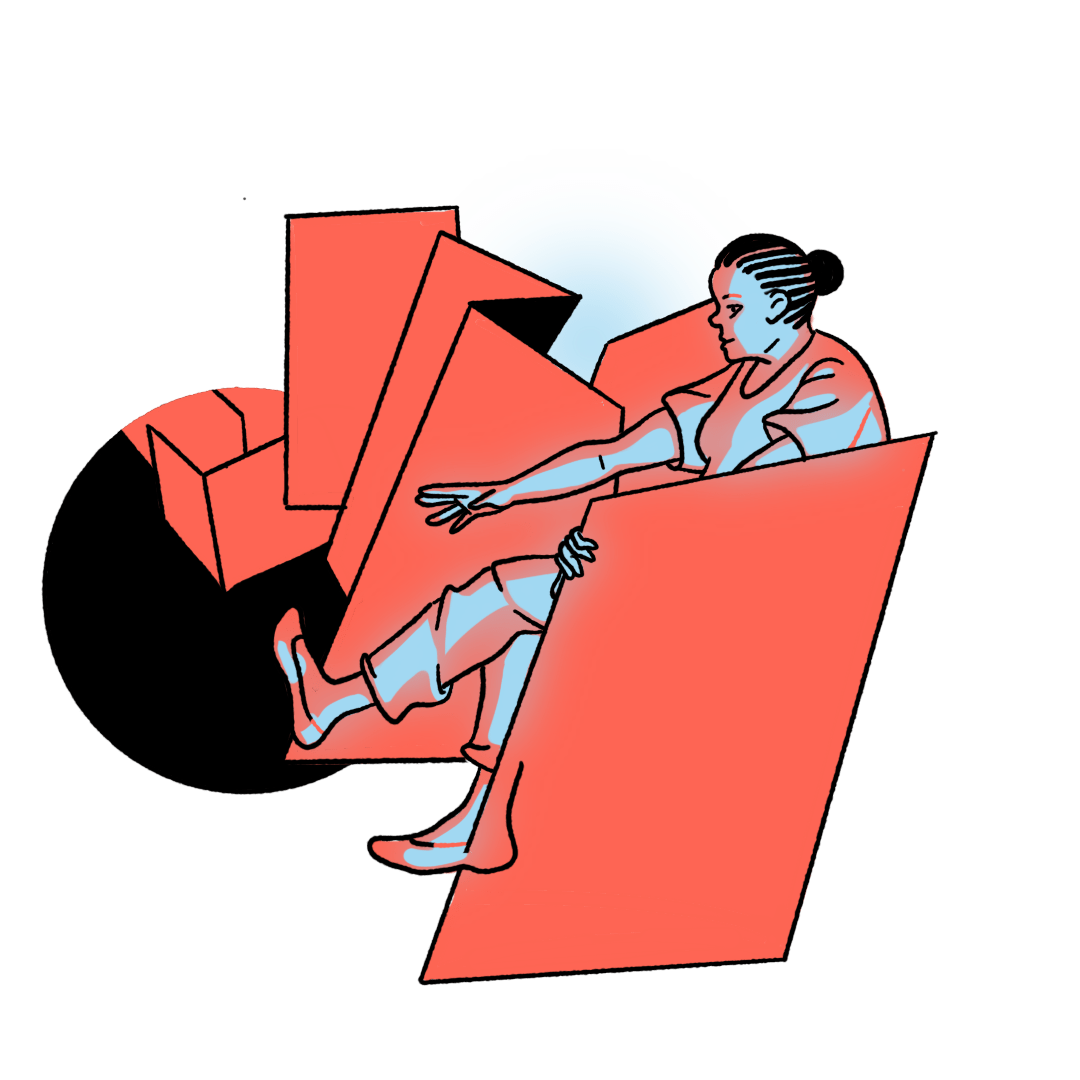
It's been a while, yes. But as you know there's no mandatory publishing cadence. Only when there's potential for great conversations.
Speaking of great, for this edition we're joined by Joe Paton, Director of Neuroscience Research at the Champalimaud Foundation.
We discuss the interconnected topics of behaviorism, learning, language models, differences and similarities between the brain and artificial intelligence, the role of responsible AI in healthcare and much more.
50 minutes that flew by for what was an extensively interesting conversation. And also the first in-person talk since the launch of this project.
Enjoy.
- Lawrence
Joe - And it's called the Learning Lab because I mean, if you ask me, what's the sort of core thing I want to understand, it's how animals kind of learn to behave adaptively in complex dynamic environments. A big part of that is how do they infer structure? How do they infer the cause of relationships between things, right?
And a large part of that is just based on what they've experienced. Right? If you give a certain set of experiences that has a certain kind of statistical structure then the brain will infer something where maybe it's not there, right?
So for instance, there's classic examples from experimental psychology where early on in training, in particular, when you're training animals on these, you know, tasks that are in Skinner boxes animals will develop kind of peculiar patterns of behavior. And they're often called superstitious behaviors.
If you go and look carefully, the behavior, you find that, "Oh, they just happened to be behaving in a certain way when they got their first few rewards" and then that seems that those rewards kind of stamped in that behavior. It's like the animal thought that it was that weird thing they were doing that actually got them the reward, right?
So then they repeat it. And then once they start repeating it, they're enforcing that structure again. And then, the whole thing can kind of snowball and then you get these very peculiar behaviors. Eventually they tend to extinguish, but when you have that incomplete data set then you do this kind of faulty inference.
And you're like, "Oh, that weird thing (If you're a pigeon) when I was flapping my right wing that's what got me the reward". No, you know, that's not...
L But we do that as well, right? We do the same thing.
J Yeah, exactly. And again, I would point to some of the behaviors of algorithms that have only had access to kind of incomplete data sets as sort of reflecting the same fundamental principle.
L Right. So you were telling me about the lab, which by the way is under the Champalimaud Foundation. Can you tell me how did you end up being the principal researcher here in Lisbon? How did that come up?
J Yeah, so I was finishing my PhD at Columbia, New York. I did my PhD with non-human primates. I was actually recording activity from the brains of macaque monkeys as they were doing simple kinds of learning tasks and kind of doing a lot of analysis, relating neural activity with behavior.
And at that time, when I was about to graduate, I was looking around at the kind of positions that gave researchers some early independence. Sort of like an independent postdoc position.
I applied around to a number of different places including Cold Spring Harbor, which is where the founding director of the neuroscience program at the Champalimaud Foundation was at the time. It was a guy named Zach Mainen.
He was working with rodents – rats and mice – doing the same kind of work that I was doing in my PhD, in terms of relating neural activity to behavior but in an animal model organism where you can start to get more information about circuit mechanisms. There are all sorts of tools in mice in particular, but also in rats for labeling specific genetically identified cell types for manipulating neural activity.
And so he was one of the people who was at the forefront of using these animal model organisms to do the kinds of systems and circuit computational neuroscience that I was interested in and he was being recruited away.
He said "We're gonna have these kinds of independent positions here at this new neuroscience Institute in Lisbon. Would you be interested in coming and checking it out?"
And I sort of thought: "Oh, okay." You know I always found the idea of living and working abroad kind of interesting. I thought: "I'll come for five years. This is kind of like a postdoc position. And then I'll establish a research program and then go back to the US".
And as I got here in 2008 we started building the program up from a handful of labs, started hiring new principal investigators, built a graduate program, built science outreach programs and moved into our new building when it was finished around 2011.
Pretty soon, there weren't any other places in the world that had created an environment that was better for the kind of work I was doing.
Then of course, living here is great. I mean Portugal is a fantastic country in terms of the natural environment, the climate, the food, cost of living – which is changing. But it's still a fantastic place to live.
We have a foundation that has kind of stood strong in supporting both fundamental research and also their clinical efforts but not trying to repurpose fundamental research into applied research, but rather they had a vision of kind of putting the two next to each other and trying to promote crosstalk between the two.
As time went on I started to get more excited about that more general vision. And so now, as you know, we're planning on trying to take that general vision in the new, specific direction that touches on a lot of the issues. I think we'll probably talk about today.
L That's definitely the ultimate place that I want to go to. But tell me a little bit. I'm curious about how such a lab gets put together and what were some of the struggles that you encountered while doing that, whether that's finding the right people or red tape or bureaucracy.
What were some of the things that were hard to bypass to get to where you are now?
J I guess one of the general difficulties for anyone who's trying to set up their own lab is that you kind of are selected to have your own lab on the basis of work you've done as someone who works in a lab, right? Doing science. And then you get your own lab and all of a sudden you have to be a manager.
There's certainly a lot of struggles in trying to figure out how to best be a good manager – both a mentor to people in your lab but also how to manage projects. Suddenly become a fundraiser, right? So you're writing grants – we've done pretty well with writing grants. We have some core funding from the foundation, so relative to a lot of places I've been pretty lucky. But those are some of the struggles and it's a constant learning process.
L I guess that's something that anyone suffers from. From going to more you do what you love, your passion and now you gotta grow it. So you can't just keep on being in your corner doing what you love. You gotta oversee, you gotta coordinate, negotiate, whatever that is. It's part of growing.
J I feel like when I talk to my friends who maybe went more say the business route it's sort of more common like: "Okay, you work for a little bit and then you go back to school and you actually get training on how to be a manager". That doesn't tend to happen so much in science. There are little courses here and there but it's just not embedded in the culture so much, you know?
L It's interesting. In a way, you don't have that learning to scale – how to properly scale a scientific organization. It's maybe more common in tech centered or business centered, entrepreneurial centered type of things.
J
There are other challenges that are kind of more on an institutional scale. We started off with like 20-30 people and now we're close to 500 people in research at the foundation. A lot of things change in terms of processes that work for organizations of those different scales.
We're still dealing with those kinds of growing pains and trying to figure out how to meet those challenges.
L And in that growth of people you guys are attracting from everywhere in the world? Can you just hint a little bit on what's the diversity?
J I think overall we're about two thirds Portuguese, one third international. We have a doctoral program in neuroscience that's about 50/50 Portuguese/ international. From the very beginning – and I think this comes all the way from the top of the foundation – there was a desire to maintain a really international atmosphere.
I think that was really important for developing our own culture because I think there's a tendency – if you haven't been in some of these traditionally very strong academic, scientific research institutions, you know, the Harvards, the Columbias, places like that – there's a tendency to be kind of like awed by it and kind of want to copy what you see as the markers of their success.
By bringing in people from all over the place who actually came up in those kinds of institutions, what you had was people who actually saw an opportunity to do things differently. They didn't think: "We just have to get to the point where we're doing it like Harvard" or whatever. We're a completely different kind of organization so it doesn't really make sense to compare the two. There are a lot of things that I think don't work about those places and beyond that we're a small foundation and so our goals are different. That should lead you to think kind of differently about how you approach problems.
L Well, speaking of goals, I would be interested that you could tell us what those goals are and then use that as segue to how the AI part comes into it?
Because I guess you started to apply AI to help you achieve certain goals, I guess, to some extent? And so when this angle of being careful and how you apply AI and what are the ramifications of it. But first talking a little bit about what are the goals, so that we can also get a context of how then this perception of being responsively applying AI comes into play.
J For us, as a neuroscience program that's been really focused on the neural circuit and the computational physiological basis of behavior, there's two different kinds of deep connections to AI.
One is more fundamental and conceptual. One way you could describe what we're trying to do is we're trying to kind of reverse engineer or understand how natural intelligence works. There's a kind of natural connection between approaches in artificial intelligence for those who are trying to understand natural intelligence.
So a lot of the models that we build to try to understand neural circuits borrow from or are really overlapping with models or algorithms from artificial intelligence. As you know, a lot of the most powerful, modern AI algorithms are based on artificial neural networks, right? So right from there, there's a connection.
And then there's more of an applied connection, which is that in our experiments, we are collecting more and more, really, really rich, high dimensional data sets for which approaches from machine learning, artificial intelligence are really powerful just for gaining insight into the data we're collecting. And so we adopt these things as tools as well, just to analyze data.
Now, risks. The risks on the fundamental side are, I would argue that, a lot of albeit very powerful artificial intelligence algorithms, they're really not replicating the way natural intelligence works. Because for the most part the tasks we're putting to these algorithms, they're nothing like the task that was put to organisms in the evolution of natural intelligence.
L In the sense that they're too narrow? They are too specific?
J Yeah. You know, the brain had to solve the problem of controlling the behavior and physiology of an organism in a complex and dynamic environment, right?
When you train a large language model you have fundamentally different tasks that you're putting to: that you don't have the same kind of continuous control problems, the real time demands. You don't have to worry about survival. You don't have to worry about reproduction. You don't have to worry about working in groups. There are all sorts of different pressures that the brain faced during evolution that, for the most part, we're not throwing at these algorithms.
So I think as powerful as they are and as eerie as it can be to interact with them, they're not yet kind of getting there by the same path that organisms do.
Going back to the risk question, if we actually succeed in building artificial general intelligence, in a way that's based on natural intelligence, then you're gonna have a lot of ethical questions.
L Is that even achievable with the hardware itself in which these models run? Considering that, don't you maybe need that those models kind of are in a body and maybe even be biological to some extent so that you can replicate what you want, which is human consciousness?
J But of course you have people who are taking deep reinforcement learning approaches to robotics, right? You've got people who are working on neuromorphic computing. There's a really big difference between the computing architectures we use to implement and run modern machine learning algorithms and our brains.
One principle difference is that we basically put computation and memory storage in different places, right? That's not how the brain works. The brain uses the same kind of wetware to do both compute and storage. So that's a really big difference. But there are actually people who are, you know, building different kinds of computing architectures that do something that's closer.
Actually I'm about to write a grant that involves (a collaborative grant) that involves working with someone who develops neuromorphic chips and someone else who works in robotics.
Because I think that if we're really gonna understand how behavioral control emerges from neural circuits, we need to go beyond building the kinds of models that we build right now, which are really kind of abstract, non situated, non embodied. Not subjected to those same real time demands and on computing architectures that are really fundamentally different than the ones that the brain is using.
L So having said that, how does it make you feel when a couple of weeks ago, there were a lot of articles coming out about this misrepresented situation at Google that we were, we were pretty close to...
J Yeah. I'm sure you guys paid close attention to that.
L Well, we were looking at it like another media hyperinflation of what's going on.
J Yeah. As I talk more and more to the media, I understand a little bit more what their goals and objectives are. So it's easy to see how these things can spin outta control.
But like I said, I think my current position – and I'm always open to change my mind on my current position – is that as convincing as it can be to interact with some of these models they're doing more... guess what I would call kind of emulation more than like simulation of intelligence, right?
They're really, really good at capturing rich deep statistical structure.
L Like an advanced parrot of sorts.
J Sort of, yeah. I mean, they can clearly do de novo generation but when you think about how the brain is operating, we already have kind of good ideas about how you can take a relatively simple algorithm, chain it together a few times and you get to something that looks like an abstraction, right?
One thing I often think about is how much data these large language models have been trained on, right? It is so far beyond the amount of data that my seven year old has been exposed to in his entire life and there's still the kind of pockets that these models kind of miss in terms of his capability. So there's clearly something different about those two learning systems.
L You won't be able to brute force it by giving it all the data in the world.
J But take, I don't know how many tens of thousands of years of experience from a human to get the same amount of exposure.
L But the thing is that you don't need that exposure.
J Exactly. There's a gap there in terms of the underlying mechanism that's producing what we perceive as intelligence and I think that gap points to the fact that it's not the full stack of intelligence that we have access to.
That said, I think it does a great job of replicating some set of layers of things that we do and obviously on the back of a lot more data than we ever have the opportunity to learn from.
So it's not like it's completely off base. There are things that it's capturing about how the brain processes information, it's just not the full stack.
L I wonder when you're talking about the full stack, are you also talking about the unconscious part? You mean like all of those mechanisms that we still don't understand how they operate and what their function is?
J Yeah. So my own research is kind of moving in a direction of viewing the brain at a more global level and thinking about the fact that it's a huge parallel processor.
You know, the brain at the end of the day is for control.
It's for controlling the behavior and physiology of an organism in a complex and dynamic environment. Nervous systems have been on this planet for 600 million years or so and during that time evolution has come up with a whole host of different mechanisms, like at a circuit level for producing some kind of control, right?
So we have in our spinal cord, in our brain stem, we've got circuits for relatively automatized forms of control, you know, reflexes, pattern generators for things like walking, movement primitives. And those kinds of more automatized forms of control can be modulated, selected, chained together, by descending input from more adaptive control centers that are more anterior in the brain.
And so you have what I call a heterarchy of control mechanisms, because each of these brain systems has a very stereotyped circuit architecture which suggests that they kind of contribute some core computation to the functioning of the whole system. But they also operate on information at varying degrees of abstraction from the immediate physical world.
So you have this kind of heterarchy and this hierarchy in the brain, a lot of parallelism, a lot of modularity, right? And so when you look at a large language model, it just doesn't contain any of that complexity and it doesn't need to control anything.
L Yeah. When you see this sort of news about the consciousness of that Google model... Ask anyone that is in the field and they will tell you that it's not the case. At best it's a very great mimic of a behavior. Doesn't mean that it knows or that it's conscious in any way.
J But I actually see this thing happening in humans also. Now we're getting more into kind of sociology, but I feel like there are ways in which you can approach life.
Which is: "Okay I'd like to take a career. What are the defining characteristics of success". Right? And then I'm gonna optimize for those defining characteristics. That's like mimicking, that's like the emulating approach, right?
And then there's people who go through what I would call a more substantive route, which is they're kind of doing the work every day, they're interested, they're motivated by the problems they're working on. They work well with other people. They're trying to learn from their experience. They're not trying to optimize for these indicators of success but those things come naturally through a more substantive route.
This is one way that I meant that comment that I said before, that I think these models actually do reflect something about the way our brains work, because we can take that kind of mode of operation where we're just trying to mimic.
L And how did you become sort of interested in this observation? What was the thing that kind of triggered your attention to those matters?
J I think it was my own experience in trying to understand how the brain works. I was trained as a biologist originally but as time has gone on I've steadily become more and more attracted to more engineering heavy approaches, mostly because we do experiments and we collect data, so we make observations and then we generate hypotheses about what those observations mean.
But for me, I never felt comfortable stopping there. I felt like I wanted to build something.
L Now I wanna do something with this.
J Yeah. I wanted to kind of provide a litmus test for those ideas. If I'm gonna go and I'm gonna interpret some experimental observation as meaning something like: "the brain is using this signal I just saw, to do X", well I should be able to build a model using that kind of "signal that does X", right?
And maybe if I build that model, I'll be able to understand something else about factors that I wasn't observing in that experiment. And then I can go into the brain and look to see whether I see those kinds of things, right?
So models that make predictions, which you can go test on future experiments. And in the process of kind of moving in that direction, you started, you know, learning more reinforcement, learning, building reinforcement learning models, right. And then you sort of get a sense for how these models work and how they can produce adapted behavior. And then deep learning comes along and you get this incredible capacity to do representation learning and things like that.
You know, just kind of in that back and forth between thinking about the brain and building relatively simple RL models. I don't know, I just saw a lot of commonality between how these models work and aspects of how the brain functions, but also kind of seeing the gap between the two. And I think the major gap has to do with this thing we were talking about, which is, in large part, we're not asking these models to do what brains are asked to do.
L I wonder how that will come to be possible. I have no idea how that's implementable as of now.
J You know, we have 80 billion neurons in our brain, right? Each of which can make up the 10,000 connections. I guess the largest models now maybe have half trillion parameters, something like that? So you know, those numbers I just gave with the brain, are ignoring all the extra dimensions of biological complexity that brains possess in terms of subcellular, molecular level of things like that.
So you probably can't... I don't think we're gonna be able to implement something that is really like at a molecular scale like the brain, but some of that you may be able to abstract away. And I actually think in the not too distant future, you may start to see things that are getting close.
L And let me ask you this. What's the usefulness of it? Tenerating that we're talking about, this ultimate God's mode achievement that we replicated, we created life. Is it like a feat or, you know, how would we then apply that? In our society?
J You know, first order, the thing I'm interested in is understanding, right?
I started interacting with these kinds of models in the pursuit of understanding how the brain works. And I think our adaptation as human beings is to try and understand the world around us. That is something that evolution has kind of endowed us with. And we don't know where the applications are always gonna come from when we build a kind of body of knowledge.
But clearly we don't get to any applications without first understanding how the world works. So I think there are a lot of different ways that this could go in terms of applications.
Now, specifically, you build like a truly intelligent agent. What's the application of that? Let's talk about the more social good, you know, like assistance for people who really need support. We're gonna increasingly have an aging population. You're gonna start to see diseases of aging in higher and higher frequencies. It's an enormous burden, both economically and in terms of human costs you know, caring for people who are aging, right? So that's one example where you really build a truly intelligent agent.
L And do you think that the moment we get to a point where it's equivalent, does that puts us in question in terms of how special we are or not? Because sometimes there is this... It's a hard problem until you solve it and then it's like a given, you know? Yeah. And AI suffers a lot of that.
J Keep moving the goalposts.
L Yeah. Like when we beat computers, we'll get to that point and then you beat it. It's like, well now it's nothing. So what's the next stage?
And the ultimate stage is to mimic ourselves a hundred percent, right? And once you get there, I would wonder where that leaves us as humans?
J I don't think of humans as being particularly special in some sense.
Obviously we're special in the sense that we've managed to build all this, like sit here, having a conversation and have it recorded using some set of transistors and, you know.. I think a lot of that though actually just comes from culture, the ability to actually save information over generations and build on it.
One thing I think about a lot is the fact that modern humans showed up 250,000 years ago, right? Agriculture only appeared 20,000 years ago. So 230,000 years before we figure out agriculture. I mean the same brain for all intents and purposes, right?
So we tend to put a lot of the power on the architecture of the brain, but actually a huge amount of the power is in the accumulated knowledge that millennia of human society and culture has generated. And if you were to wipe all that clean between today and tomorrow, it would take a really long time to get back there.
I think it's sort of embedded in your question that if we were to make this AI that completely replicated our abilities it wouldn't be so special on its own. It would be special if we gave it access to all the accumulated knowledge t hat millennia of human society has generated.
L Yeah, that's true. It's true that one of the main factors of our success is definitely the ability to tell stories and pass them along generations and the language as well.
Complex language is an amazing technology of our brain. I mean I don't think there's any species that is as complex as ours in terms of speaking. No. So all of those have given us advantages and then we were able to do all of this. Build things and create things. Think in abstract manners.
J But even that language, you know, sure we have some inborn tendency and capacity to use language but, again, wipe all the languages away from today to tomorrow, what does language look like? How long does it take to spring back up again? And in what form? I don't think we really know.
L But the thing that is fascinating is that it emerged, right? AI is something that you're consciously building. Humanity has a pocket of people trying to build it, but the brain itself – evolution – makes it that there is a mechanism that allows for language to emerge at some point in history. So how does that emerge?
J Well I was saying that one of our specializations as a species is trying to understand the world around us, right?
L Do you think that's something that we kind of have in us?
J Yeah, definitely. I think so.
L On a genetic level to some extent?
J Yeah, it's got to be. Definitely.
L The curiosity to look for and explore.
J Yep. I think that there's an inborn drive to understand. I think it has led to the development of a lot of alternative explanations for how the world works throughout history. I'll leave it at that. And we lost a lot of other things that might be useful for some species to survive like physical strength. We are not particularly amenable to extreme temperature changes. We had to use our brains to come up with ways to allow us to populate every corner of the earth.
And another thing that is an adaptation of a subset of organisms on earth is that they work in a social context, right? So because we're weaker, because it takes such a long time for us to develop – because we've got these massive brains – it was critical that we work together in social structures, right?
And once your survival and propagation of genes becomes dependent on working together in social structures, now you need ways to communicate, right? Because that's only gonna make those social structures more powerful.
L So in a way it would make sense that that would evolve and become stronger.
J Yeah and you can see these sorts of proto languages across the animal kingdom. They maybe don't have all the kind of grammatical complexity of human language, right? There is a gap there for sure, as far as we know, but you can see proto language is all over the place.
So I think the same pressures that led to the development of those pushed the development of language in people.
L Interesting. I mean I would just be talking about evolution and the brain but I wanna bring you back to the technical part, the tech part, which is the main audience.
I think we can speak openly about the Responsible AI Consortium which is something that both Unbabel and Champalimaud are working together with many, many others.
And it is an interesting time, I would say, because we are attempting to do this internally in this country, but many other countries have realized that we got to that point where decision makers are knowledgeable enough and the examples are visible enough to say we need to kind of maybe be on the lookout on these technology, because it is so impactful on a wide scale on so many areas. That we can't just blindly install these black boxes which are proprietary many of the times, and who knows how they are being developed. And do we truly understand them?
So many ramifications that you see these efforts to kind of start talking about ethics and the responsible usage of AI?
Well, actually Microsoft – I think it was yesterday or two days ago – they came out and said that they would limit the usage of some of their facial recognition. So those are some signals of private entities coming out and saying, we don't want that to be misused.
So can you tell how the foundation yourself, you guys view this effort being led? I mean, with, or without the Consortium, right?
J So maybe it makes sense to tell a little bit of a backstory and how we got to actually first getting to know you all here at Unbabel and the next things we want to do and how that fits into the Responsible AI Consortium.
So around 2019, I became director of the neuroscience program. And at that point I asked the foundation administration what do you see for the next phase of the neuroscience program? And they said very general things: that we'd like to see connections between research and the clinic and we always like to see new technologies being integrated with what we're doing here.
And I don't know if everyone that's listening to this knows, but the foundation has a cancer treatment clinic and we're a fundamental research program focused on the neural basis of behavior, right? So the connections were not totally obvious.
If we were like a molecular biology department then there might be links with cancer biology and so on. So we had to go back and sort of think about what would represent substantive links between our neuroscience program and clinical activities.
And we sort of came back with two approaches. One is to build up capacity in machine learning, data science for all the reasons I was just mentioning about the connections between neuroscience and these areas previously, both fundamental and applied. But also because on the applied side the areas you can apply these tools to are really, really broad. And of course there are a lot of clinical problems that are ripe for machine learning.
Okay. So that was one thing we said. The other thing we said is you could broaden your clinical focus to include more explicitly human behavior.
So fast forward a couple years COVID hits, we're kind of in a holding pattern for a while. But as we're sort of coming out of it we realize we want to build connections to the local community in sort of more engineering machine learning areas. That's how we first got in touch with you guys.
And we began to kind of develop this vision for this new center that we're gonna build at the foundation, which is focused on what we're calling human neuroecology and digital therapeutics.
Now one way to think about this center that we want to build is that it's sort of like an organism, right? An organism has to take in information from the environment. It has to kind of analyze that information, gain insight into it. And then it has to use that information to take some action, right?
And so on the input side of this center, the idea is we build up this machine learning data science capacity to take in various different kinds of data in the health space that would be patient data or data about people, you know: clinical samples, imaging, behavior and then try and feed that into the development of interventions that focus on behavior to improve health.
And for a lot of people that might be a strange idea. "What does he mean for health?" Well if you think about it, let's focus on disease for a second: it's genes, it's environment and it's behavior, right?
90% of the healthcare burden worldwide is chronic disease. So it's things like diabetes, obesity, cardiovascular disease, various lung conditions, mental health, stroke, cancer. Okay.
If you look across all of those things, behavioral factors are some of the largest determinants of risk and prognosis. And in many cases, whether you can actually reverse the disease process.
And we said "Wow! Given that fact, why aren't we as a society, investing more in trying to use what we understand about neuroscience behavior, to use all this capacity to collect and gain insight into data to research and develop interventions that'll operate on a behavioral level? To change people's behavior or to give them behavioral protocols that are gonna help them recover or help them improve functioning?"
So that's kind of the backstory. That's an overview of how we're trying to build up a kind of applied research setting next to a fundamental one in this area of health.
But it's gonna rely on dealing with a lot of data, right? And this is data about people and their lives. It's really private data about the inner workings of their bodies, you know? And so when we started to dig into this issue and realize everything that's involved you realize several things.
One is, obviously, this is sensitive data. People have a certain inalienable right to lead an autonomous and independent life, right? You can't just go and take things from individuals. We decided as societies that this is in conflict with our sort of morals and our ethics.
But the other thing is that there's actually a lot of social good that can be done using data science, using machine learning. But it requires enormous amounts of data...
So how do you maximize the social good with these kinds of approaches while being respectful of these values that are so important?
And then you start to realize that... We're just not prepared. We're not prepared for dealing with these issues. The way that we've tried, I think at a regulatory level to sort of protect the interests of individuals and their privacy and things like that. But in the process, what we've ended up doing is basically locking a lot of data away in ways that limits the potential good that you can do with these approaches.
And so fixing that problem – I'm not saying that we need to do away with those regulations because they're really important – but I think there are ways you could basically change the system and the way we treat data that both gives individuals kind of more control over their data while at the same time lowering the barrier for feeding data into these kinds of tools so that you can maximize their benefit.
So that's one of the areas I'm really interested in, in the things that the consortium's focused on.
There are also specific projects. I don't know how much you want to talk about. I mean the brain project here at Unbabel.
L Oh yeah. Well, honestly, Paulo [Dimas] would be a much better person to talk about it. My involvement in it is kind of like what this podcast tries to do.
So one thing that I thoroughly enjoyed listening to you say is the sense of responsibility that a scientist or technologist has in their work and the respect for the subject of their work, which in this case are humans and then trying to actually grapple with those constraints.
And one of the reasons that I started this – and I really like the consortium idea – is that it's a way to basically create some sensitivity towards other scientists, towards other technologies whomever is developing something that may be deployed and applied to a part of society, even if it's a small part of society.
So that's why this project to me was instantly appealing.
And by the way, the thing that you guys did, the Metamersion is, I think one of those things that you can totally do and bring the audience and expose them... well, in that case, it wasn't necessarily like risk usage. It was actually very beneficial with the game.
But it's actually unlocking this curiosity towards the public or future researchers, future grants applicants, whomever, in combining that into how they solve problems.
And I think we've gotten to this place. That's why you see more and more... The European Union and the United States and other countries, these forum of people saying: "We need to find a way to handle this".
Either that's with consortiums and boards of people that oversee and that create legislation and some way of controlling that. Or getting into those institutions and private companies and kind of nurturing this mindset from within.
J I think that the latter approach is really important because the way I see things going is that... you know: the only constant thing is change, right?
We're constantly gonna have to be vigilant and we're gonna constantly kind of have to check our current moral and ethical principles against the most recent developments. And the idea that we'll be able to just kind of define a set of rules from the outset that are going to be kind of timeless it's likely to not bear out because things are gonna change.
And then we're gonna have to react to that. But the important thing is to kind of be clear on what the principles are and then be vigilant and constantly update.
And so the more you can build these things into the cultures of the places that are adopting and developing these kinds of broaches, the better, I think.
L One of the main struggles of people that want to bring ethics into the realm of technology like startups and tech companies, is that when there's no buy-in from leadership, because it's kind of like a secondary thing to the OKRs then it just gets put on the side.
And the question is: it can be okay, you know, the outcomes are not that problematic or they can be, which is what we've seen since those bigger models have been deployed over the last 10 years. And then you need to react, right? So putting a bit more time and consciously trying to anticipate some of those outcomes.
I think that that's one way to start. It's a combination but if it's only enforced by some external agency then it's never gonna be as good as it could.
J Again, I take a kind of reinforcement learning approach to a lot of things. My work influences the way I think about strategies in general.
I try to look for ways that you can create an alignment between what is the kind of morally and ethically correct approach to a problem and optimize whatever is the incentive within the system you're in.
So if it's like you're in the health space and you want to maximize your impact on health and wellness, right? Well, this example of data and how we deal with data, I think there are ways to basically both give people control of their own data and free up data such that it could be fed in at a larger scale to these kinds of methods, thus maximizing the power of those methods.
But right now we don't do that, right? Like you go in, do a clinical trial, whatever. Your data gets collected and then that center, that university, that hospital locks it down in order to comply with regulations basically. With good reason, right? But you can imagine a world where everyone has their own data repository and we've got protocols and infrastructure and processes and institutions to advise people on how they can kind of distribute their data.
L I've read about it, yeah. Sort of decentralized "you own your own data".
J Yeah. And I don't think it's like a panacea, like it's the thing about decentralization, it comes with its own problems, right?
L But do you agree with Google kind of like making partnerships with specific labs and partnering with medical institutions, getting their data in order to see to what extent they can apply within their own models... That's okay?
J world where they have to make a deal with the entire health system of Singapore or whatever to get the amount of data that's necessary to actually kind of start to ask interesting questions about their methods.
But what I'm saying is that I think there's maybe a future world that is a little more aligned with our principles in terms of ethics where you don't have to do that.
Where Google can go, look, we have a call out for these types of people and send your data here via this protocol X, whatever, we'll have access to your data for X amount of time. And there's a whole system set up for selecting which aspects of your data you're willing to share and to whom and for what.
So you kind of build in to a totally new system. You have a way of basically getting data fluid enough that you can have it at a large enough scale to actually develop approaches.
L Do you use Apple health?
J I do.
L What do you think about it?
J Again, this is my own personal position on it. I wouldn't wanna project it onto anyone else, but basically I don't worry about it. Like in terms of them having access to my data.
I, myself haven't encountered a situation where I'm so concerned that they have access to a lot of information about me. But I would never wanna say that, you know...
L Maybe Apple would be in a position of having enough data to then use it and apply it in developing something, I guess.
J Right. But I think in an ideal world...
L It shouldn't be a private corporation to do that? Or..?
J Well, no. I mean imagine: Apple's an enormously valuable company, right? And if a significant fraction of their value is on the backs of the data of individuals, then you can imagine in this new system, new ways of writing contracts, right?
Where you go like: "Okay, we can have your data, but if we make x amount of money some fraction of it gets returned to you", or something like that. So there's a way to like, build value back into it and "Here's the things we might use it for".
Actually they probably have a lot of that stuff written in the agreements.
L Yeah. Minus the money getting back to your part.
J Well, but there's no good way to do that right?
L They can send it to my Apple wallet.
I use it [Apple Health] extensively and I like the gamification and I try to, you know...
J Yeah I use it all the time. I look at mine, you know, heartbeat.
L And I'm hopeful that maybe Apple with their narrative that they do want to care for people's protection and, to some extent, they're working in that sense, that ultimately that could benefit me, you know?
In a couple of years, maybe 10 years they do have partnerships with medical centers. And you can look at this whole data and try to understand why you are in a certain condition or not.
J Yeah. Yeah. So there's an example of collecting data at scale. Right. But it's only certain kinds of data, right. It's not very deep.
You can actually learn a lot from it, I'm sure. But I'm talking about things like let's look at a whole bunch of circulating biomarkers, you know? Let's look at a high speed video. So when you start to add in all these layers then maybe it gets a little more sense of meaning. It gets, I think, more complex. It gets more complicated.
Yeah but I'm more than happy to take that deal where I get to gamify my own health and Apple gets to take my data.
L That's the trade off in this case. Which we gladly do, I guess.
Listen, it's been super interesting. I look forward to see what will come out of the consortium and definitely keeping an eye out on research and the work coming out of Champalimaud as well. Cuz I do understand that you guys are basically gonna go with this approach one way or another.
That's the way I understood it and that makes me glad, you know?
J Yeah. And again I think there's a lot of fertile ground for developing new approaches, both for applications and for developing a fundamental understanding of things by starting to get companies, people who are developing products to solve certain problems, talking to people who are doing fundamental research so I'm super excited about it.
In addition to the subject matter of the consortium which is – for many reasons, not just the ones I mentioned – really, really important. So yeah, I'm excited.
L Great. I appreciate your time and hopefully we'll get to speak again.
J Definitely, no question. Thanks a lot.
L Thanks.
J It was a real pleasure.
"Unbabel leads creation of Responsible AI Consortium" by Mafalda Freire [Portuguese Only].
"OCEAN CAMPUS: the new project that brings together the Port of Lisbon, the Calouste Gulbenkian Foundation and the Champalimaud Foundation" by Champalimaud Foundation.
"The Uber files tell a simple truth: democracy depends on curbing mercenary tech giants" by Rafael Behr
If you've enjoyed this publication, consider subscribing to CFT's monthly newsletter to get this content delivered to your inbox.