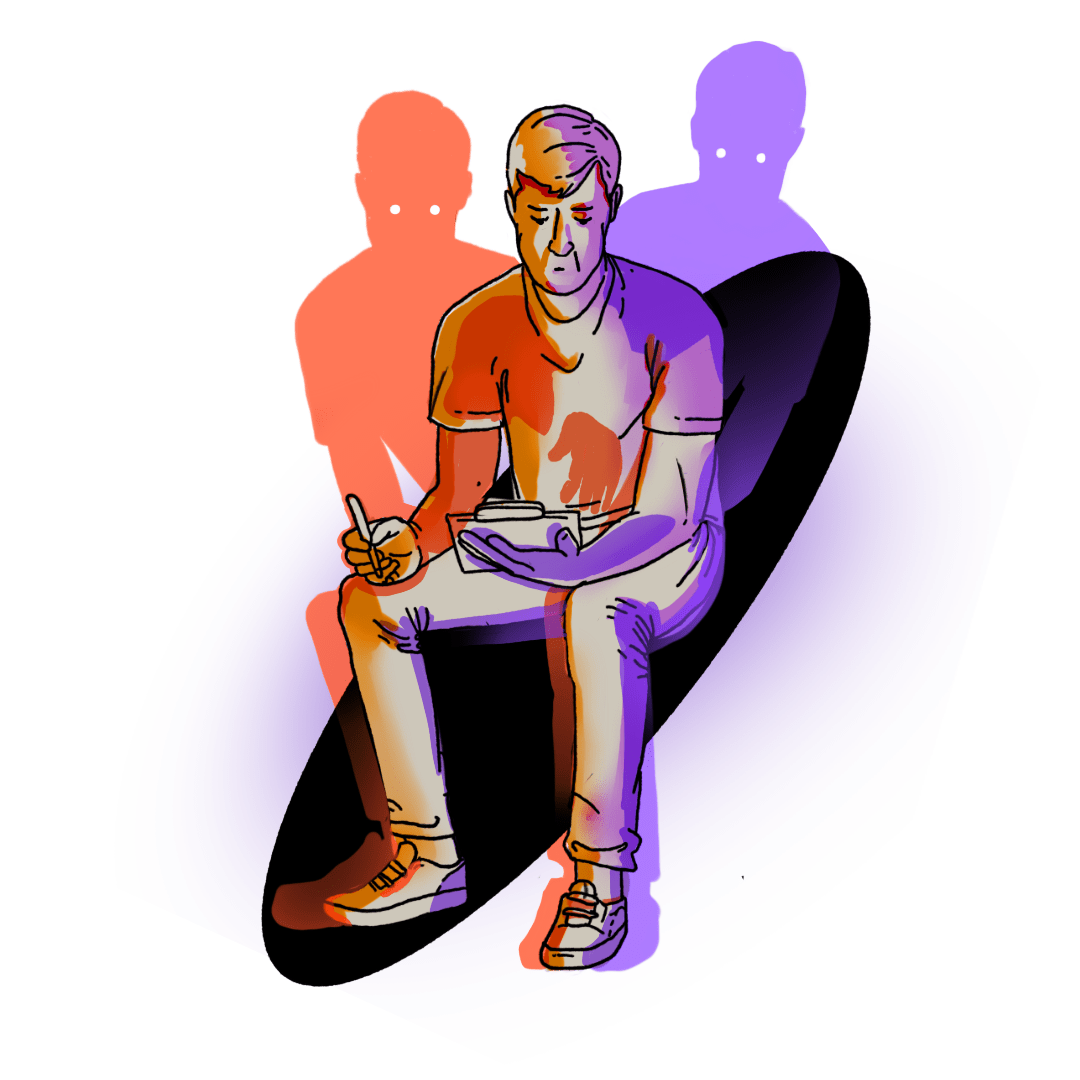
There's always a first time for everything. In this case it's the first time an issue is delayed! Attempting to multitask can pay a toll... As per Mozart's words: "The shorter way to do many things is to only do one thing at a time."
For this 13th edition, Unbabel's CTO and cofounder João Graça joins us for a wide-ranging conversation on the human-AI relationship and the future of work, the difficulty of doing machine translation and the possible dangers of AI
- Lawrence
Lawrence - Hi everyone. Today we have João Graça, the cofounder and CTO of Unbabel.
João has a PhD in natural language processing and was a postdoctoral researcher at the University of Pennsylvania.
He's also one of the cofounders of the Lisbon machine learning summer school, has authored several papers in machine learning with side information and supervised learning and machine translation.
And also, as a disclaimer, being the cofounder of Unbabel, he is my boss. So I must disclose that I work for Unbabel as well.
Hi João, welcome to Critical Future Tech.
João - Hello Lawrence, how are you doing?
L I'm doing fine. So on this Monday morning, rainy Monday morning, one of the main topics that I told you I wanted to discuss with you is something that in society, when you have an AI powered company – a company that uses AI – one thing that comes to people's mind is that AI is going to take over the world. It's going to replace us all. It's going to take our jobs.
Sometimes, because we (Unbabel) essentially provide translation services supported by AI, people think that we are just replacing translators, but that's not really the case.
What I wanted to discuss with you is this dichotomy between AI taking over our jobs or AI supporting us, and showing that we are actually more towards the AI supporting humans instead of replacing them, right? I guess over time, you've had this conversation with many people, right? Can you tell us a little bit about it?
J So that is a fundamental question that has been asked about Unbabel, but also about society.
Let's start with that. So the first thing is that there's a problem. People speak different languages and they want to communicate and right now there's two options. You either assume that people know all the languages in the world, which is not feasible, it doesn't happen. Or that everyone will know at least one language, which is pretty much English, like the lingua franca to communicate, or you need to have some translation in the middle.
And so translation has been growing a lot. And for instance there was a study, I think four or five years ago, that if you took all of the professional translators together, they could not translate 1% of the content that was being produced. So there's not enough manpower, there's too much content being produced at every given day to have translators.
So on the other hand, a lot of the content that is being produced is very simple and repetitive, and you don't need a professional translator. So for instance, you're a native Portuguese, like I am but we are talking in English and we write emails in English so we're not kind of like professional translators and we can do this translation job.
So the premise of Unbabel from the beginning was that we can use non-professionals translators to do translations and basically increase the amount of translations that you can do massively. And by this the side effect is you're actually bringing a lot of jobs for non- specialized persons.
Now at the same time, AI has been improving so a lot of the content that can be translated can already be translated with AI. And so let's do it. Let's make the goal of humans being able to communicate with each other a real goal and say that instead of just 1%, we can all do 70% and AI is going to make the most of it. Now, the question is whatever is left is still bigger than 1% so you're not removing jobs from humans.
There's another question which is: as AI progresses, are we going to get to a point where AI can translate everything? I am not a believer of that. Especially in the coming future there are some types of content that are very hard for AI to deal with.
AI doesn't know how to deal with, you know, hidden meanings or metaphors very well. So AI right now is a very good memorization machine. So you can read stuff and can memorize it and spit back. But it can do a lot of, like, emails. They are always the same. We don't need humans.
So there is a trend of: what are humans translating, that is going to be from everything, to kind of more complex things. And so for instance, at Unbabel, the way we work with people is on one hand to translate some data for us to train the machine and the machine gets better. And then basically we identify the areas where the machine is failing and to have the humans go there and correct these steps and fix them. And trying to make the machine better.
But we will still have more work for people to do. So I don't think that anytime soon you're going to be out of job as a translator. I don't have data to support this, but I'm quite confident of this statement: there's more work for translators now than there was ten years ago, five years ago.
L And that's because of market expansion? Companies going into other places? Or the remote workforce? Why is that?
J So first of all the internet is becoming more and more global and more trade is being done through the internet. And there's a lot of studies that show that people feel more confident in buying stuff if it's in their own language.
You're creating this internet in different languages. People are producing local content that needs to be translated. But then it's like, once you make it available for people to translate some part of the content with machine translation in a much simpler way, then it's a barrier to entry that becomes smaller.
So this is kind of like where we are right now in reflection. Now I think that over history, we always saw that technology tends to remove some of the existing jobs and create other jobs, which are normally higher cognitive load jobs.
So for instance think about agriculture. The amount of people that you need in agriculture is completely different than the one that we needed 50 years ago.
So there were a lot of jobs that would destroy agriculture, but you don't have more unemployed people than you had 50 years ago, you just created other jobs. So I think there's going to be an increase in the level of cognitive load that people will be doing work, which in fact I think is good for humans.
And then obviously if you keep pushing this topic further, you start getting to the point where, well "what happens if AI does all of this grunt work?" Well, then maybe people start having more time and that's where you're seeing all the discussions about the basic income for their work, which I think overall is going to be very good for us as a human species.
L We're still to see that, right? But that does seem to be the trend: that more and more we'll be able to eventually spend more time doing more intellectual work, creative work, instead of doing the repetitive and cumbersome work.
On the language side of things, a couple of months ago, I was reading this book that I really enjoyed by a Portland State University professor, Melanie Mitchell, on AI. And she basically recalls the story that initially some interns were recruited like in the fifties. I don't remember who the professors were and they were naively saying: "okay let's try to make a machine translation program during a summer internship".
And that was like 70 years ago. And still nowadays when you look at systems like Google, DeepL and others, ours. They are good enough to a certain extent but at some point they become kind of dumb because again, it's more than just translating words. You need to translate meaning and machines still have a hard time understanding the meaning of things.
They can translate the sentence, but they don't really know what that means. And when you meet a translator and when you know how to translate, even if you're bilingual. That's when you see how hard translating can be because of the meaning of words and the context that machines lack nowadays right?
And that's why we actually need someone, a human at the end of that, to actually see if the meaning of the translation makes sense, right? Not just the words themselves, but the meaning of what is being conveyed.
J To me it's semantics. I would say the same thing, but there's also all the cultural things. Like, are you conveying the same sentiment? That's something that is very hard for machines to do, for several reasons.
First of all, the models that we use are actually limited. So our models only look at one sentence at a time. So everything that you need from the previous sentence to disambiguate, you can't do that.
But also again we're not very good at taking these cultural nuances. Because if you think about one of the grand evolutions that happened on machine translation, there were several neural machine translations, but one of them was word embedding.
So it would be the case that you basically took one word and you look at the entire corpus and you say: "Okay, this word means this". You used to do it with just counting, counting some features. Then you have these vectors of representations. But for instance, one of the things that we had an issue with is that there was no way to solve this was words like "bank". "Bank" can be the financial institution, it can be the place where you sit, it can be the part close to a river. And so the algorithm had to kind of understand what it means based on two or three different words, but it was the same representation for that word, although there were different semantic components.
Now, with this new BERT model and word embedding this is not the case because you basically take the vector of a word, given the sentence where it's connected so you basically get different representations. You can see how even this already gave you a boost. But still, if you think about everything that we know about the meaning of a word in the current society where you live, for the age group that you are in, you know that there's a lot of dimensions of the word that you can't capture by this. It's just.. It's in your brain.
And so that is very hard to capture by a machine. So you need people to do that work. You know, the current systems, they fail for complex stuff. They're super powerful for easy things. And there's a huge amount of things that are very important.
So let me give an example that I was overwhelmed when I learned about it. We had a project a couple of years ago with Microsoft, Dublin university, Translators Without Borders, that was about crisis translation. So the idea was how fast can you train a model from a language pair that is normally very low resource and you can use a community of editors such as ours to bootstrap data? And when I say fast, you needed to get the model in one or two days.
What was the use case? So when there was an earthquake in Haiti it was a huge disaster, a lot of people were dying, a lot of people in the middle of the disaster that need to be rescued. And so a lot of countries send people there to help them, called first responders.
Now, they spoke some French Creole that was not easy to understand and the first responders were coming from Germany. All countries that couldn't speak that language. And this is actually people on the field that will tell you that they would go there and didn't know how to interact with the people that need help.
"Who is worse? Is it that person or that person?" You can't understand. And so Microsoft did something which is to create some bootstrapped models for the language pairs. If you look at the actual quality, it was terrible, but it was enough for people to start communicating and that made a huge impact.
So how can you do this with this technology? And that is actually saving lives.
L That's a very, very interesting example of deploying AI in this case for the societal good.
J So that was like a super interesting story. Now it raises a lot of interesting questions. Imagine that you're an editor of Unbabel and that you're sitting at home on a relaxed Monday holiday doing tasks and then suddenly the tasks we get to translate is of someone that is below two meters of dirt, dying. And you feel that if you don't do a translation fast enough or you miss, the person will die.
And so, the question is: "What kind of people can we use"? Do you need to train them psychologically? There's all these interesting questions that came up that I never thought about. But those are examples where even the most basic technology, because when this was deployed in Haiti, it was not even neural machine translation. It was like the previous iteration of systems whose quality was much worse. [It] already brought a lot of positive impact.
I think with the technology that we have, we can cover a huge portion of the needs. Now, the last 20%, those are always going to be harder. And if you're trying to translate like a Dostoevsky, then yes: machine translation will not do it anytime soon.
L Do you think that at some point AI is going to be able to translate a whole book as we were giving the example of Dostoevsky and, if yes, then how long do you think that would take?
J Okay. So I don't have an answer that tells a plan like: "I think it's five years because ABC is happening". I think we're still in the belief phase.
L You're not convinced yet that it's for the next 5 to 10 years.
J I mean, I think it's possible. I think we've been learning and improving a lot of things very fast.
But for instance the current technology in AI, everything that you hear about the big language models that's actually, for me, a really dumb approach. It's like a brute force. It's just putting a lot of engineering to make these models that are basically a super good memorization machine.
Now, one thing that has been taken for granted is that you can't memorize all the knowledge in the world. Even if you have super big models. Maybe I'm wrong. I was seeing the data set that they use for this new Microsoft model and it's huge. You need to have some reasoning. And the first thing you need to do is you need to have an abstraction, a semantic abstraction of the world.
And this is where a lot of research has been going. So we haven't progressed on that. Our abstractions are super basic and the research that you're hearing about, at least in the media, it's not about a better understanding of abstractions, it's about more volume, more memorization. But then you're just memorizing stuff. You don't know exactly what you're memorizing, what to pick.
And I believe that you might be able to translate a book with these systems because the book is just a bigger thing. The problem is in the nuances. There are nuances that you won't be able to get, and that will happen either in a book or an email because we don't have the proper reasoning mechanism.
I can't predict what we're going to do in 5 or 10 years because there's an exponential growth of technology that we've been doing. I don't think that the way that we're progressing towards that will solve this problem. I think that needs to be changed. And when that change happens, we might get closer.
Besides the lack of understanding of the actual structures, we still use these pretty basic models because of the computational limitations. There's still a lot of work that needs to be done for us to have a model that could potentially solve a translation problem.
What I'll say is that we'll see translation becoming much better. And we'll see a lot of use cases just using direct translation. Not only because it's becoming better, but because people are more willing to take machine translation errors and live with them than they were before.
So there's a compromise on the need to communicate.
L People are like fault-tolerant on the quality of those systems, but it's one of those super hard problems, it's funny. Sometimes the media doesn't do any favor because they're like "GPT-3's here and it's able to write whole articles". And then you go try it and it's very fantastic, but if you actually try to make it write without any human intervention, you'll just be laughing because it makes no sense.
Even if it constructs really interesting paragraphs, it's unable to keep its "line of thought" about the subject that you wanted to write about, which it deviates from. Some random tangents really well-written could be someone (a human), but it doesn't make any sense. It's tricky.
J And that has been one of the biggest issues with AI. You know, these computers that learn and will dominate the world. And then if you ask the practitioners, we're so far away from there, like we can't even envision how to get there. And there's a lot of really interesting talks about these. So for instance one talk that I thought was really interesting was about why AI wasn't dangerous.
Because AI is just trying to do a task. If you were able to implement a system that could self-reproduce physically and had the goal to basically survive, then it might become dangerous. But you're not training any AI to do that. You're just training AI to do translation.
But when you have this hype, people start having a lot of expectations and this always leads to the AI winters. There were a few of them. But then suddenly there's no investment and everything is bad. And you know, the thing is there have been so many things happening over the last 4... let's say 10 years, that a lot of applications of AI became actually useful to be used as a product they use.
They usen't to be used as a product and now they are. And at the same time, there were developments that put AI in the hands of everyone. So AI is becoming a commodity.
And I don't know if you remember, 4 years ago, all the hype was about chatbots. That was completely hype, we're not there. Because we don't know how to map the intent of a conversation and the consequences. So what you have is, you have glorified template systems like you have for the last 40 years.
They're still very useful by the way. The only part that is not true is that you have an agent that understands you and talks with you.
L That's a big switch case as I like to call it.
J It's a smarter switch. Let me give you another example: machine translation evaluation. So the new systems, like COMET from Unbabel or BLEURT from Google.
Before you had met measures, that would be lexical. So they look at the word, length of the word and measure how many words appear and are the same. This was BLEU. BLEU, METEOR, those have a lot of problems like, for instance, you could be saying the same thing with different words which made an appropriately correct translation: it would score zero. Or, you could have a translation with many similar words, but had the word "not", so completely different meaning, be very close.
So these new systems, because they don't do this, they take it to the semantic space. So they represent a sentence as a vector. So similar sentences are set to show similar vectors. So now these new systems can capture those things much better. You know, that was the progress.
L It's always very incremental. It seems that there is never like this crazy leap forward that you go 0 zero to 10.
J These are super hard problems. So this was only for the ability to relate to things, semantically that we do as humans, that was a leap forward.
And by the way, we don't know how we work ourselves. So we don't know how the brain works to even be able to model it.
L Or if we even have the right hardware to mimic our own hardware, biological hardware.
J I think that that will be easier. I think we can build better hardware than the one that we have here, I think it's the abstractions that we don't have.
L So, let me ask you something. What you were talking about, which is, the dangers of AI: is there a danger or there isn't? So if there is, in your opinion, what are the dangers of AI? If you have thought about that already.
J So I don't think there are dangers. I think there are opportunities. I think we're living in a world where we, as a society, can determine what we want. So let me give you an example. I don't think there's danger because I don't think there's any self-replicating AI system that can go and start creating their own society and decide they want to eliminate the human race.
There's nothing. They're not programmed for that. They do learn how to do one task, but I think it's very unlikely that the goal's system is going to decide that he wants to replicate because it's a computer. So let's remove the danger part because honestly, I don't think there is this.
Now what we have is we have a lot of AI systems taking daily decisions on the spot that can impact human lives.
L Yeah. It's a more realistic danger.
J Yeah. It's like self-driving cars. Or for instance, once we start going to war with drones that are controlled by AI, you know, it's not that the drones are going to revolt against the humans, but they're going to be making calls at a single point like "Who do we kill? Do we kill that person or do we kill that person"?
That is where I think they can make wrong choices and we need to be careful. How do we program those? What is your loss function? What is the error from missing that thing versus missing that thing? There's also an opportunity because for the first time we as a society can codify this. Because AI will not make individual choices in the sense of an individual like me, I'll make my choice on the spot. I'll make a call myself and then you will make a different choice. So each one of us has this ability to make our own choices.
When you have AI and you have 10 drivers, it's not going to be João or Lawrence who's driving. It will be the same AI that's reading from the same program where we as a society have codified. In the event that you have to kill one person because there's no other choice, that's a hypothetical event. And one is white and one is black, which one you wanna kill?
L One of the arguments of the danger is that AI can perpetuate certain stereotypes or certain biases within society right? That's been a lot of the conversation in the past 5-8 years, right?
So I guess what you're saying is that it will replicate what we do as a society, but then it can keep on pushing certain things that we don't actually want to keep on pushing, right?
J I'll get to the bias later. So the first one I want to make is that you now have a centralized point to make decisions, which we didn't have before.
If you ask the question like: "I have an older person, a younger person, the car has to drive (over) one. What do you do? I have a terrorist with a kid in their arms, but with a bomb. So if I kill the terrorist, I might kill the kid but I won't kill 500 people".
You know, all these things.. There's a point, there's a centralized point that's going to make a decision. You have control there. Until now you didn't. So that's where I think lies the opportunity.
Now the question is: I am saying these as if you could just write rules for every single situation. You can't. A lot of the rules are inferred from data and that's where the bias comes, right?
That's almost like an entire new episode: what to do with the bias? So I think that again as a society, we have the chance to remove some of the bias and removing the bias is easy. So that is the easy part. You can just look at the data, you can compute some statistics, you can see where the bias is coming from, and you can just add or change the weights on the learning algorithm, or you can replicate the data that you want to "unbias" and voilá. You have an unbiased dataset. So I think that is the easy question to answer. The hard question is what bias should society want to remove or not?
I was actually having a conversation with a friend about this and... Okay let me get through the controversial example which I don't know the answer to.
Let's say nowadays, you're a financial institution in a city where the black population is actually poorer. And so if you give a loan to a black person, you are in fact more likely to default than you're not. Okay, so this is the current situation. And now you have an algorithm that tells you exactly that. So there's a bias there. Now you might decide, or someone might decide that it's not fair to have that bias.
So you want to remove the bias from black people having a higher interest rate because they can default more.
First of all, who gets to make this call? Is it a committee? Is it the country? The state? Is it the bank?
Now when you make that call – this is an example, it's not the reality – if it's true that black people default more, when you basically put that constraint on the algorithm you're making the financial institution lose money. You're making a societal decision that will make a private entity lose money.
This is an example. It's pretty easy to say "Yes, but we need a positive bias, so we really want to do it". But then where's the limit? If you assume that you want to remove all bias, then basically what you're telling to financial institutions is like "You need to give credit to everyone without looking at anything". It's a flat rate. It's a 25% flat rate for everyone. But then what's going to happen is instead of some people having low rates, everyone's going to have the same rate and then the institution is going to start protecting itself.
And this, by the way, this happened in the UK with car insurance. They removed a feature that was discriminating towards, I think it was older people. And then what happened was like a lot of younger people didn't have money to pay the insurance, the car insurance, and they stopped having insurance with it became a problem.
So these unintended consequences of removing bias.
I think there's a much more deep conversation than the one that I'm reading a lot on the news about some of the biases. Because yes, they exist. Yes, some of them aren't fair. And we as a society have the opportunity to change it. Now, the question is where do you stop and where do you begin? You have to make a conscious call about what you want to remove and how you want to remove it. Does this make sense to you?
L It makes total sense. And it's a very hard question.
It's a question that the European Union is interested in knowing how to answer, right?
The thing is most if not all AI systems nowadays are deployed by private entities, right? It is private entities that have the most cash and the best researchers and the most, the most to gain from, right? Because they want to productize these solutions and then deploy them and they do so being a private entity.
So first, who is within those companies asking those questions. There aren't a lot of these companies with people asking that. You have the bigger ones, right? You have the FANGs, the usual suspects that, to a certain degree, will allow you to question it up to the point that it starts hurting the margins. And it's understandable, it's a business. But then the question should AI only be deployed when it clearly has a positive benefit to society. Right? So you can have an image recognition system that, regardless of bias, is looking at people on the street and is assessing threat levels depending on whatever data points the system decides to use, right? Your dress code, your gender, your age, whatever. And then you have the same sort of AI image recognition used to detect cancer.
So two very different applications of the same principle, but the purpose of that deployment is very different. Then who gets to decide, "You can deploy this, or you can deploy this"? And in my perspective, that's where I think we're getting there right now.
Let's pay a bit more attention to what systems are being deployed. What are the sort of outcomes that they generate and whether or not we want that to be put out there in our society.
But it needs to be, in my opinion, the governments, the institutions to kind of have this role to ask those questions and then maybe develop some regulations, some boundaries, otherwise we know what's going to happen. Private entities will want to maximize profits. And I think the danger of AI is precisely that. It's that you deploy it but then who controls those systems, right? Who gets to make the call at the end of the day of what is a bias and what isn't? And if it's very opaque, because it's private, so you don't have access to it.
It's a hard question for sure.
J So there's a lot to unpack on your statement. Let me just go back a little bit to where you started. So you started about who gets to decide and I agree with most of what you said. And this is a problem that has been solved before, you know, there's a lot of technologies that were developed that required some intervention. For instance, nuclear energy. There's a nuclear energy committee that decides who gets to produce nuclear bombs, nuclear power plants, because people realize that this is too dangerous to be used. And I think we need to have something like that.
Now, let's make no mistake. That is not fair, right? That committee is run by the most powerful countries, four or five. So, replace country with institution and you have pretty much the same. Take the example of Iran. Why can't Iran have nuclear power? Because someone who has nuclear power, who has nuclear bombs, has decided that they can't.
Now, I don't think there's a possibility of a world without something like this. This will always happen. So the same thing is going to happen to AI. Is it the companies? Is it a country? Is it the UN? Like how much do you control what people can do?
One thing that you hear a lot is like all this Facebook calamity. Like, what Facebook can do, what Facebook cannot do. And for me, I kind of understand the point and I think they could be more careful with their algorithms.
But one thing that people tend to forget: no one is forced to use Facebook. Facebook is a private company that offers a public service that people use on their own will. You don't have to. And then what they do is they sell advertisements. And the way it is monetized is by the number of times that people are looking at ads.
And so they are training an algorithm to maximize the time that you look at an ad to make some money. Now you might say: "Well, but they could do it for a better purpose. They could put things that are less controversial."
L If you leave it to companies, and you look at some of the history of companies that have business models that end up being against societal good for one reason or another, right?
It's kind of hard for them to self-regulate. And so there needs to be some sort of intervention, which I think it's going to happen. Europe is definitely interested in having some answers, at least some guidelines and some sort of regulation to some extent on how you can deploy and use AI for your business model.
And then we'll see what are the consequences of that. And one of the things that I also hope is that from within companies, technologists and also founders and everyone involved is able to make a decision to not use these solutions in a way that could harm groups, minorities or society at large. So that's what I'm hoping for.
Now, how do you do that? I guess it's by having discussions, bringing people together and just trying to solve this. Because it's not going to stop, you know, it's just moving forward, but we can move forward without knowing where we're going.
J And I agree with you that at some point there has to be some external intervention because you might be so closed in your inner circle that you don't even understand the harm that you're doing.
I think the point that I was making is that this is something that annoys me a little bit. It's the perception, sometimes, that some people have discussions that these companies own everything to society when... You know, for me, there's a motto, if you're not paying for the product, you are the product and you need to understand that because there's nothing bad with that.
Why do you need to understand it? Because you can just keep using Google, Google Maps, Gmail, you can't put all your life on a thing that costs a lot of money to develop and assume that that company is not taking anything on their interest for this. And I do think that a lot of these companies are... Most of them already give a lot of stuff for free that they could be charging. And sometimes I think you put too much weight on one of the sides of the scale. Now having said that if you have too much power, you need to be controlled in some way. The question again is: who controls?
L Yeah, we can also wrap it up, I think it was already a pretty interesting conversation, but it's exactly that. It's that these things... you need to be looking out for them because it kind of creeps on people, right?
Suddenly you realize five years from now "well this technology, this solution has really disrupted society" because it's so insidious and you just accept it and you start using it. Suddenly, it's like, well, that's the way it is now. If you don't have this then you cannot like, whatever, ride the subway or buy groceries.
And so we kind of need to step back a little bit as technologists. And that's why I also wanted to create the Critical Future Tech discussions. Just like step back, have a look at what you're doing. Have a look at what you're creating, ask some questions. Don't just blindly run towards the enticing complex problem.
Solve the problem but also ask yourself: what's the application of solving the specific problem, right? What are you, where are the unintended consequences maybe? And so for that, you need to kind of have a bird's eye view, maybe of society, maybe of other people. And just say: "if I do this, which seems to be the right way, the right choice, where are the things that I'm not seeing? What are the unintended impacts that I don't really know about?"
And one of the things that I've realized by talking with people here is that you need to bring other sorts of people. If you only talk with technologists, only with programmers or only with machine learning people, your opinion on things is going to be very narrowed.
And so one of the things is to bring other people from other parts of the industry, or even different industries, creative industries, psychologists, you know, like whomever. Have this conversation around technology and just have their say. I mean, that's a strategy I'm going for here.
J That's actually very interesting, because I always think about bringing more AI experts and their AI experts to the discussion but they're most likely going to say something similar to what I'm saying. So maybe get a different perspective from people who, maybe, don't understand technology, but are impacted by it.
L It was a pleasure having you here. We need to wrap it this way. Unfortunately, the internet is not participating today. I don't know why. But I'll guess we'll just wrap it up like this.
J Alright! Talk to you later, it was a pleasure. Bye-bye!
L Bye!
You can connect with João Graça on LinkedIn.
"Algorithmic tracking is ‘damaging mental health’ of UK workers" by Dan Milmo.
"The tech billionaire aiding the Facebook whistleblower" by Emily Birnbaum.
"How Digital Powers Will Reshape the Global Order" by Ian Bremmer
If you've enjoyed this publication, consider subscribing to CFT's monthly newsletter to get this content delivered to your inbox.