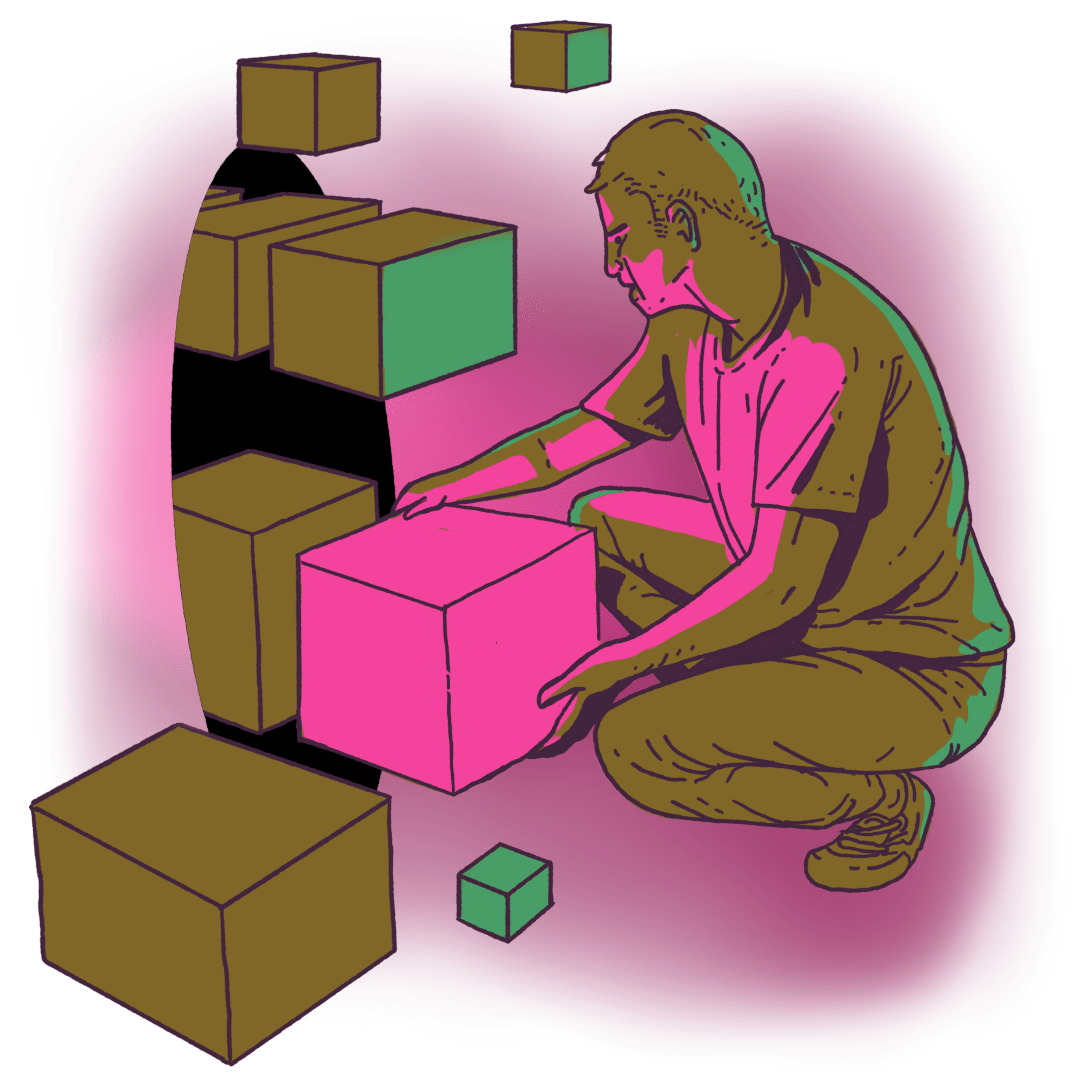
Whether that's because we're back to work or school, for many,
September is when the year (re)starts. Cleaning up is often part of
a preparation process, like before starting a new cycle.
For this edition, we're joined by YData's co-founder and Chief Data
Officer Fabiana Clemente, where we discuss the need to access
quality data, how to account for bias and how data and algorithms
can shape society.
- Lawrence
Lawrence - Welcome everyone. Today we have the pleasure of
talking with Fabiana Clemente.
Fabiana is the co-founder and Chief Data Officer of
YData, a Portuguese
company, whose mission is to help other companies and data scientists
to access high quality data to develop better AI solutions.
Hi, Fabiana. Welcome to the podcast.
Fabiana - Hi Lawrence. Thank you for the introduction. I can proceed and present a bit about my experience and also talk a bit more about YData if that sounds good?
L That sounds amazing, yeah.
F Essentially, my
background is in applied math and since the beginning I always had
this kind of pleasure to see insights being extracted from the data.
So this was the reason behind why I decided to pursue data science
as a career. But while I was having the pleasure of working in the
world of data science and extracting insights from the data I
understood that it's not as straightforward as we think. There are a
lot of problems. There are a lot of challenges and one of the
challenges I found throughout my career was related to the quality
of the data.
Of course the problems around data quality depend a lot. It depends
on the use case we are tackling, it depends on the organization you
are working for, if it's a bigger organization or whether it's a
startup. The pains of privacy and regulations, not giving you access
to the granularity or the data you need to do things in a timely
manner. Or being in a startup where the data access is there, but
you have no data available at least to tackle the use case you want
to explore. Or the data is not in the right form of the right shape
or the right inputs, because there were manual errors, there were
misunderstandings on the business definition or the problem. And
that of course led to a lot of problems being replicated into the
data.
And essentially this was what motivated us, myself and my co-founder
Gonçalo to start the company. And nowadays, as you very well
described, we have a data centric platform where we are offering
this platform as a way for the data scientists to relieve the pain
that they usually have while trying to have the best data possible
to build a model that in production does deliver value for the
business.
L And to make sure
that I understand, basically there's the model side of things, which
is the code if we want to call it that way. And then there's the
data that feeds into that model.
So you guys are tackling the part of the data where fundamentally,
if the data is not good, then the model, no matter how great it is,
is going to be suboptimal, right?
One of the questions that came to my mind when researching for this
podcast is: is there a sort of generic approach to what is good or
bad data that you can give us?
F This is my opinion
and I guess this is very personal in the sense that it's definitely
the vision I'm embedding in the company. In general it definitely
depends a lot on the use case and mainly on the business objective
or the value we want to extract from machine learning.
That's why I think it's very hard to give the ready to use solution,
fully automated, to validate the quality of your data regardless of
the use case you want to explore. Of course, there are a few things
that you can measure and it is possible to have them run in a fully
automated manner in a way that you already know if something very
off is going on with your data you can tell right away.
For example a very specific case: you want to assess whether the
quality of your labels is good because it's not always as
straightforward that you have labels. Then that means that you have
more than enough to go and pursue your use case.
So in an automated manner, you can identify what is good or not but
it might depend on the use case, the label or the target variable
that you want to use.
So these kind of things is where we believe some flexibility should
be given to the data scientists, and we believe that data scientists
should impute the business knowledge in order to get the best
information possible about the quality of their data.
L And I know that
good and bad data can be on multiple levels, right? You have good
clean data but that is biased towards one thing or another, and you
can have great clean data that is not biased, but the information
within that data is bad in a sense that you could use it to target a
group of people and so it's also considered maybe bad data? Again,
it depends on the model that you want to apply it to.
You were saying that in a way you need to adapt how you look at
data. So you have your platform, but do you help your customers? Do
you work with your customers to tailor your platform somehow? Or how
does that work since you sometimes have to have a custom approach to
things?
F One of the things
that we say, and is always important, is that there is no data
quality without knowing the use case. There is always a need to
combine, let's say a development of a model, even a baseline to
assess whether by iterating through your data, you are getting
better results on the model that you are using.
Afterwards, if you want to tweak the model that's of course
recommended, but you can work upon a baseline of quality and that
has to be based on a model.
Usually the tailoring that is needed is done by the data scientists
as if they were developing. Of course in some cases there are use
cases that are more exotic and in those ones, we do help by tweaking
some tools in order to cover those use cases.
L Thank you. It makes
sense. Especially at the beginning of a company, you're going to
want to understand how you need to evolve and even sometimes pivot
your product in order to satisfy your customers' needs, it's very
interesting.
So, to get back to the original pain points, you talked about
privacy, GDPR, HIPAA and so on. Your pain was that accessing the
data was hard because of all of these sorts of restrictions and
protections around personal data.
So it is my understanding that, somehow, you are able to transform
the data in order to speed up using it for the data scientists that
work with you? Can you elucidate a little bit on how you go over
this barrier?
F Of course, of
course. Sometimes it's the belief that the regulations are just
there telling you to not do anything. And of course security teams
do prefer to go for what is, let's say, more secure and more
obvious, which is just restrict the use [of data] instead of
exploring it a bit more and understanding how they can still comply
with the regulations and at the same time not blocking innovation or
evolution of some uses.
Of course you can do a PCA or a dimension reduction algorithm in
order to keep some privacy of the data. That's one of the
methodologies that can be used. But when you do that, you lose
explainability and understandability of what the data is telling
you. The other methods that exist are anonymization, obfuscation, et
cetera, where you just hide or you mask whatever is your
information.
We understood that data synthesis is one of the options. Of course,
data synthesis is something that is not new so it's in the market
for quite some time. The difference is the technology that you can
use to do the data synthesis.
I'm telling that: in order for these data to be used for data
science, besides the granularity, you also have to keep for the
other two main pillars: the fidelity and utility of the data,
because of course you can synthesize the data, but if it doesn't
hold the information and the utility of the original one, that means
you cannot build machine learning use cases out of it.
So in that sense and on that scope, deep learning technology ( and
not only), generative models in general are pretty good at doing
this. When we are talking about, for example
generative adversarial nets, they are quite interesting in a way that in a data driven manner
or data derived way, they understand the behavior of the
distribution of your data or the data that you are presenting to the
model. And after learning these patterns, these behaviors, these
correlations at first and second level, you are able to replicate
this into random noise.
So you just captured the relations, the distributions, and now you
apply those distributions into random noise so you can generate new
data. The fact that you are using random noise and your data-driven
knowledge on top of random noise, you are ensuring things that are
important for the privacy aspects, which are one variability on your
data.
As in everything, there is a trade-off on privacy. The more private
this data is, the less utility it has or the less fidelity. So there
is always a trade-off but essentially the same applies for the other
methodologies privacy. And in this case I would say this is
definitely one of the most data science friendly ways to ensure
privacy while guaranteeing usability for the data scientists.
L A very interesting
approach. I wanna ask you just some things before we move to the
topic of bias. So you mentioned very accurately that, of course it
is a trade off between protecting identities and the privacy and the
usefulness of the output that you end up with.
So who controls this balance between both? Is it the customer or do
you provide the customer the ability to fine tune that basically?
F Yeah, it has to be
the customer. So in a way it's upon the users to define the privacy
budget, let's say.
It is really called the privacy budget. So how much are you willing
to give up on privacy or how much are willing to keep on privacy and
how much utility can you get with the privacy budget? It's a
trade-off and it's a visual trade-off, you can really control that
to understand how much you are giving up on one in order to have the
other.
L Oh, now I'm curious. How does that work? How do you set up this budget? How do you calculate it?
F So the calculations
depend a lot on our evaluation metrics for example, for the utility
side, especially. The privacy side and especially the privacy budget
comes from also some metrics on the privacy side, but a lot from the
use of techniques such as differential privacy.
So differential privacy already brings you that interesting concept
of privacy budget. And essentially those metrics combined with each
other is what allow us to show a bit of that trade-off.
L So they have an idea of what the trade-off is going to be.
F Kind of, kind of.
L Let me see if I can
segue this into the bias part. So, in a way you guys have some
metrics and some leavers on what constitutes a privacy data point, I
would say? And I guess you can add more or less into this formula
that determines the final trade-off outputs
So I guess you use techniques like established industry techniques
to determine that. Do you also have your own sort of gut feeling?
Well, not gut feeling, but your own sensibility as to what may or
not constitute a privacy data point that needs to be factored into
these budgets?
F A lot of what we use
definitely is studied and well studied on the literature specially.
Of course, some of those we have rearranged in order to be
applicable for the types of data that we are researching. For
example, one of those is definitely time series. It's very obvious,
let's say or more obvious when you are talking about tabular data
and records that have no time dependence.
But when you are talking about time series, the notion or the
concept of privacy is not so straightforward. So definitely there,
we had to explore a bit what is in literature about privacy and
transpose a bit to the time series side. That was a challenge to be
honest.
L Yeah, for sure. You
guys still have a lot of ways to explore. Let me then get to the
topic of bias because nowadays and for some years now, bias in data
is one of those hot topics, right? " Machine learning, AI, they're
doing bad things not because of the way they're deployed, it's the
data they were fed that is bad. So if we fix the data we fix the
output."
It's definitely one of them, one of those narratives. And, in the
sense that you guys prepare data, how does this notion of bias gets
into your day-to-day reality?
And let me split this into two questions. So secondly, how does that
happen? But first, how important is that for you guys? This notion
of bias, bias in data and in the field of AI as a whole, actually.
F I guess that
definitely is one of the hot topics, but I can say that is one of
the most important ones, because in the end, when you have to
explain why your model is behaving like it's behaving, there are two
layers that you can analyze: your model, you can understand the
predictions your model is doing, getting to understand how it's
behaving. But when you go and debug your model to understand why
it's behaving like that, part of your explanation comes from the
data.
So this is what we consider as data explainability. This is one of
the most important topics that we have to explore and to monitor the
behaviors we have within data, and to be able to spot potential
issues for your business.
And regardless, bias is more and more a problem for the businesses
and it's a problem that they have to account for. So definitely it's
something that for us is core.
L So you guys help in that to your customers?
F Yes.
L Okay. Because if
you prepare data on behalf of your customers and they have an issue
that they need to explain, I guess they're going to go back to you
and say "Hey, what's up with that data?", right?
Can you tell us a bit on how that enters your day to day operations
or your strategy? What can you tell us about how that is affecting
your work?
F Essentially it
shapes a lot of the product roadmap. First it's not as
straightforward as we might think. Let's say you have females and
males and you have the same amount of females and males, but in the
end, when you go and check the amounts they earn they are clearly
not equal in that sense.
That's bias. That's bias on your data. And that's not obvious just
by checking whether you have categories that are balanced or not. In
that sense for us we have in the roadmap a big chunk of data
causality which is what we are exploring for not only the part of
the bias explanation, but also to help the users understanding
better why certain behaviors happen when they deploy a model based
on the causation that you can extract from your data.
That's how this affected for example our roadmap or our product. And
in that sense, we offer the module for the knowledge and instruction
of the understanding from the data. And afterwards we are also
working on getting mitigation solutions to solve exactly these
problems. In the first instance, we have the side of the data
synthesis to help mediate the presence of bias but we are also
working on different solution levels to solve different ways,
depending on the bias or causation problems that you might find
within the data.
L Isn't it possible that while synthesizing the data that you also transpose bias into that proxy?
F In a way it is. Let's say for example if you do have a data set and that data set has bias, your data synthesizer will mirror that existing bias within the data. But you can control that to not be replicated in your data synthesizer.
L So you need to be aware of what to modify in a way, right?
F Exactly. What to control or what to condition.
L Yeah, that is something that I wanted to ask you. So how do you understand bias because data sets are different depending on the customer, industry, the use case so I guess that there are sorts of biases that you could maybe be missing? How into this industry or the origin of the data do you need to be in order to be sure that you're not missing some sort of bias, right? Is that a valid question?
F Yeah, it is. It is.
And that's why the module of the side of the bias we give it exactly
as a framework that can be somehow tweaked or controlled, depending
on the business logic that the user wants to impose.
Of course we have a certain level of bias. So in that sense I
haven't found the perfect way to - regardless of human inputs -
identify bias. And I think that for some time we will have this part
of the bias detection that in a sense will depend on users and on
people.
And we have to, in a way, believe that the people are doing the best
for keeping the best interest for the end users that will be
impacted by machine learning.
L It makes me happy to hear someone saying that bias and this whole complexity is something that can shape the product roadmap, because it means that the product indeed wants to tackle that, and it's not some sort of afterthought. But I guess for you guys, it cannot be an afterthought because the consequences can be very direct, right?
F Exactly. In our case, it had to be from the birth or in this case, in our core. But it's also fascinating. It's a world of possibilities, but I guess it was something that we have accepted when we started the company. And, and I guess we have to keep pushing for that evolution in this area and for more investment in the area of identifying bias, causality, explainability in particular, at the data level.
L Yeah, big problems are always hard to tackle.
F Exactly.
L I want to ask you a
little bit about your feelings regarding the ecosystem regarding
data science in Portugal, but first let me throw you a really weird
question. It's something that I've been reading lately.
Essentially, in terms of having bias into the data that is fed to
the algorithms, there are two schools of thought, right? So if you
observe the world and then you extract data, and then this data
replicates bias, it means that you have observed the world as it is
and then your
algorithm will work in accordance with this observation.
So there's the school of thought that is like "use this data because that's the real world", in a way. And the other one is using data for justice in a
sense, which is, "well, if that's biased and it's a bad bias, then we should use
these and tweak it so that the algorithm actually produces a
behavior that corrects the problem that you see from the data that
you observed".
So which school of thought do you agree with?
F So that's a very
hard question and I guess very hard to explain without creating some
division on the opinions, I guess it's impossible.
I will tell you one reason why I don't like the first one.
This happened during COVID last year I think. So let me see if I
recall this. It was in the UK or something, they had decided to use
a machine learning model to do the grading of the kids at school.
And they had decided this because unfortunately, due to COVID-19, it
was not possible to do the final exams or something of that sort.
So what they thought was, "okay, let's be fair and grade everyone with a machine learning
model, based on the data that we on students". It sounds good and sounds theoretically possible. Of course you
are already understanding the problem that comes from here.
Essentially what happens is you use the data from students, but of
course, some things that have impact in your life are not easily
translatable into data for models to understand.
So what happened was students from schools that are more problematic
got the worst grades. Of course the more problematic the school, the
worse the grades. But of course you have outliers there and you have
students that are amazing and they have students who were always bad
at school, but for some reason, in the last month, they had better
grades, which means that something changed in their life and they
started doing their work in a different manner.
But the fact is that data was used as it was collected from the real
world.
L "As is", right? So as it [the world] was.
F And we see that this
was not fair and not even true because what I understand from that
line of thought is that if you are using the data as it is from the
real world, you are not going to penalize anybody because in
reality, you will just stay the same.
So that's why I don't like the first one. The second one I like
better, but I think there is a fine line between what is correcting
the data, what is fair and what is justice.
I think it's very hard. You also put your life hands in the hands of
judges to define what is fair and what is just and we see they
commit errors. And I think there are no, you know, perfect
solutions.
But I think we will commit a lot of errors in defining what is
justice for the data. We will make a lot of assumptions that will be
wrong and prove to be damaging. But I think we have a long way to go
in order to identify what is, you know, correcting the problems in
data collection or the problems of the world, because the problems
will exist. And the issue is that the data already comes with those
problems because it is the mirror of society. But also what is the
point of keeping society the same? So you want this one, but I think
we have a long path.
L Well, I am
definitely on the second option, even though I totally agree that
who determines what is fair and where is that limit and who changes
and how is that changed?
I guess there are two. One is a very technocratic sort of, "let the
algorithm show you what is the thing? But then it's non-human,
right? And the other one is like "no, actually technology must be
used to improve human lives and if the outcome is just things are as
they are then inequalities for instance, will never be changed
because the algorithm cannot know how to do that, right?
So technology must serve humans. And so I'm definitely on "team
human" in that case.
That sort of conversation is interesting to have, of course not
practical for your day to day work, but it is interesting to think
about that because in the long-term, all of those small choices and
ways of looking at things will compound. And that's why I'm talking
with data scientists and technologists, because we are the ones that
end up actually doing that with our hands, you know, like
programming stuff so it's cool that we think of the future, right?
F Exactly.
L Let it be for the
good at least.
I wanted to know what is your feeling in terms of these
conversations happening? The sort of high level topic that we were
just having now in Portugal?
Is that something that you end up seeing as being a topic of
discussion with your peers or with your colleagues or outside of
your company? Or do you think it is too early or uninteresting? What
is your opinion on these sorts of topics in the Portuguese tech
scene?
F I think that in the
Portuguese tech scene we see more and more of these topics coming
up.
In some cases I can understand why these topics might not be, you
know, on top of your mind, it's not the core of your business.
You have so much to deal with, but I'm also happy to see a lot of
the big companies and also specially on the startup scene that do
show this is a concern, this is a reality, this is something that we
are investing in.
And we see that of course ourselves, at YData, we are assuming that
role, but you also see companies like Feedzai doing the same and
others in the market, which is very good to be seen.
And I think that definitely has to come from the company itself
because the data scientists are learning from the culture of the
company. It's where they are working. And the more the companies
motivate these kinds of discussions to show this is important,
although it's not core business, this is something that we have to
think about.
This will create a certain ethic on development that I think
whenever a data scientist is designing a solution, we'll think about
those when developing or designing or implementing. And that's why
these discussions are so important and we already see those
happening. I just think we have to see them more often.
L Yeah, I agree. It's
not the sort of thing that you want to be thinking... Well, I would
love to be thinking about this all day, but I don't get paid for
that. But it's definitely conversations that I think we need to
start having on a more frequent basis and that's why Critical Future
Tech is here to also push forward these sorts of conversations.
Fabiana, thank you so much for sharing everything that you just told
us today. I'm really happy to have had this conversation. I really
enjoyed your answers on that curveball question that I sent you. I
hope you enjoyed it, thank you for your time.
Let us know where people can follow you? Where can we keep up with
YData's work?
F Lawrence, thank you
for inviting me for the podcast, it was really amazing. These
initiatives are always important in our country's tech scene.
Regarding where you can find well at
https://ydata.ai, but
we on
Twitter
and
LinkedIn.
But I really recommend my personal Medium which is
@fabiana_clemente, where you can follow our recurrent data science topics and where
we explain more about data synthesis.
You also have the
Synthetic Data Community, our
GitHub
which is open source. And stay tuned because we have also an open
source [project] to be released about data quality.
L Allright guys, you
heard it. Stay updated because some goodies are coming out.
Fabiana thank you so much and I hope we meet again and talk again
one of these days.
F Of course. My pleasure.
L Bye.
F Thank you. Bye-bye.
You can connect with Fabiana Clemente on LinkedIn, Medium and Twitter.
"Artificial Intelligence: A Guide for Thinking Humans" by Melanie Mitchell. An overview of artificial intelligence and how people tend to overestimate its abilities.
"There’s no escape from Facebook, even if you don’t use it" by Geoffrey A. Fowler. You pay for Facebook with your privacy. Here’s how it keeps raising the price.
"Artificial Intelligence Incident Database" - The only collection of AI deployment harms or near harms across all disciplines, geographies, and use cases.
If you've enjoyed this publication, consider subscribing to CFT's newsletter to get this content delivered to your inbox.